How can I optimize my credit card fraud outlier detection process?
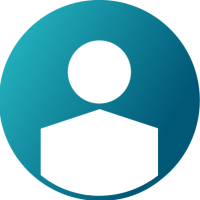
Hello everyone,
I am very new to rapidminer. Im currently working on a process in which I wish to detect credit card fraud with one of the detect outlier operators. I have found the best success with the Densities operator. I have taken a sample of 1000. The denseties operator finds there to be 381 outliers and 619 not outliers. Actual amount of Fraud is at 83 though. How can I optimize my process, so there are not as many transactions getting flagged as outliers when they aren't fraudulent? I am aware that maybe a different operator/ process could be more efficient but I am tasked with operating on the detect outliers. Any input would be helpful, thank you very much!
Explanation of steps: Numerical to binominal to change "fraud" to true/ false, Set role to put fraud as the label, sample the size to 1000, normalize the data, cross validation with decision tree to see how it does with deciding on true/ false, finally detect outliers (distance) with distance 1.0 and proportion 0.95 and squared distance.
The data set i use.