High Accuracy, low recall and low precision - how to optimise this?
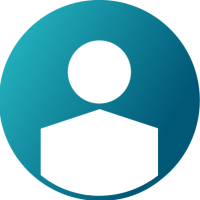
lord
New Altair Community Member
Hi experts,
I have a dataset with about 40,000 data and would like to do a classification. I have a binominal label (yes/no). To create the model I take a decision tree. Then I want to apply the created model to a training data set (30,000 data) via the operator Apply model.
Overall I have a very high accuracy, of almost 94%. But my problem is that the class "no" has a very high recall (98%) and a high precision (94%). The class "yes", on the other hand, has a recall of 7% and a precision of 19%.
I work with the Optimize operator (Grid). I also use Cross Validation as a sub-process. Furthermore I work with the Performance Operator (Classification) and I have already used accuracy and kappa as main criteria.
I know that there have already been similar questions here in the community, but unfortunately they haven't helped me yet.
Really looking forward to your help & thanks already upfront!
Tagged:
0
Answers
-
Hi,first I would consider to move away from a Decision Tree and try a Random Forest. Your Decision Tree is likely a small one, which mostly predicts " yes" and only in rare cases predicts "no". You are bias towards the majority class of your sample.Afterwards you may consider to tune your threshold using the respective threshold operators.BR,Martin0