How to get F_score in Naive Bayes sentiment analysis
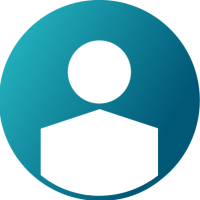
HeikoeWin786
New Altair Community Member
Dear all,
I am getting an error when I connect the performance matrix (binomial) to the model.
I need to calculate F_score as my datasets is imbalance..
Will be truly appreciated
if anyone of you faced this issue before or can suggest me the way out here.
thanks a lot in advance,
regards,
Heikoe
I am getting an error when I connect the performance matrix (binomial) to the model.
I need to calculate F_score as my datasets is imbalance..
Will be truly appreciated
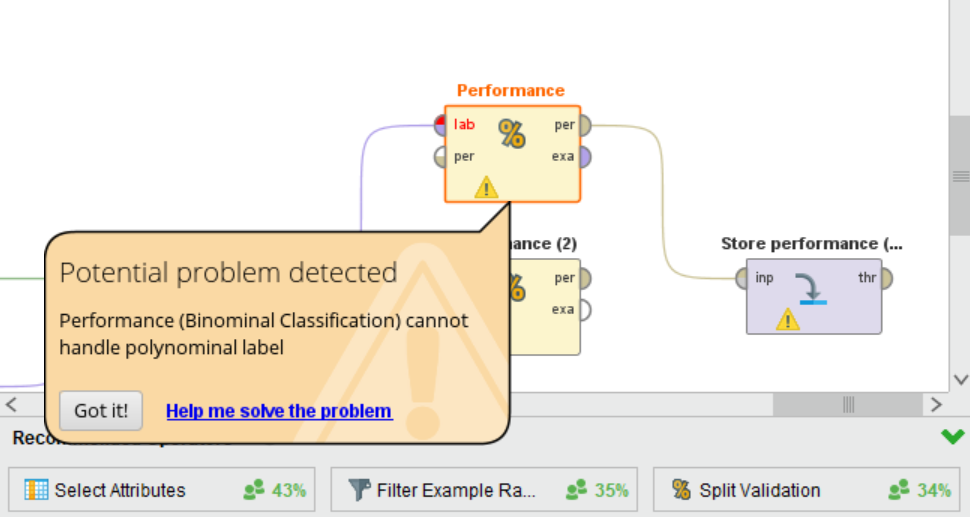
thanks a lot in advance,
regards,
Heikoe
Tagged:
0
Best Answer
-
Yes, pretty much spot on, you can balance the training set to avoid the model bias but you apply the model to a test set which has a mix of label classes which is representative of the population. Just ensure that if you do any pre-processing for training you will need to do exactly the same pre-processing for a test set (except the class balancing) , using the pre-processing models from the training run (you can save them and then retrieve them later).
5
Answers
-
This error is telling you that your label is polynominal (meaning it has many potential values) and not binominal (meaning it has exactly two values). So you need to make sure you are using a compatible label for this performance operator.
1 -
You can also use a normal classification performance and measure Kappa, which also better copes with imbalanced class distribution. However, any model trained on an imbalanced label classes may end up biased towards the majority class, so performance measurement may not fix your problems. Instead you could try balancing your classes before model training, e. g. using SMOTE operator, and then apply the resulting model to the test set which has the original class distribution (to get a realistic idea on the model performance). Also always check the whole confusion matrix rather than a single value performance measure.-1
-
@jacobcybulski
Hello Jacob, thanks a lot for explanation. For this, if I understood correctly,
1) Retrieve training dataset --> SMOTE --> Pre-processing the data (Process data to doc) --> NBC --> Store the model
2) Retrieve training dataset --> Pre-processing the data (Process data to doc) -->apply the model (which we stored in step 1)
Am I correct?
thanks much,
Heikoe0 -
Yes, pretty much spot on, you can balance the training set to avoid the model bias but you apply the model to a test set which has a mix of label classes which is representative of the population. Just ensure that if you do any pre-processing for training you will need to do exactly the same pre-processing for a test set (except the class balancing) , using the pre-processing models from the training run (you can save them and then retrieve them later).
5 -
@jacobcybulski
Fully understood, Jacob. I will try as advised. Much appreciated for your time and help.
Regards,
Heikoe0