Find more posts tagged with
Sort by:
1 - 4 of
41
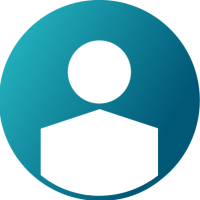
MPB_
New Altair Community Member
Accepted Answer
Hi @asiddiq , you could use the RapidMiner operators to manipulate the data so that they are in the way you want and then use the "Execute R" operator to execute LIME
https://cran.r-project.org/web/packages/lime/vignettes/Understanding_lime.html
What clustering methods do you want to apply?
@asiddiq
Are you trying to use regression clustering together for predictions ? This can achieved by utilizing Hierarchical classification, wherein a clustering algorithm breaks your data into clusters and you have regression models for each clusters. Thus, ensuring different sets of data have different models.
For model interpretability, you can use Explain Predictions as @mschmitz suggested, the operator can suggest global variable importance and impact on model base output, as well as, local results of attributes supporting and contradicting the model output for every row. You can also check out SHAP in python, which is a game theory based model explanation package.
Are you trying to use regression clustering together for predictions ? This can achieved by utilizing Hierarchical classification, wherein a clustering algorithm breaks your data into clusters and you have regression models for each clusters. Thus, ensuring different sets of data have different models.
For model interpretability, you can use Explain Predictions as @mschmitz suggested, the operator can suggest global variable importance and impact on model base output, as well as, local results of attributes supporting and contradicting the model output for every row. You can also check out SHAP in python, which is a game theory based model explanation package.
Sort by:
1 - 3 of
31
Hi @asiddiq , you could use the RapidMiner operators to manipulate the data so that they are in the way you want and then use the "Execute R" operator to execute LIME
What clustering methods do you want to apply?
@asiddiq
Are you trying to use regression clustering together for predictions ? This can achieved by utilizing Hierarchical classification, wherein a clustering algorithm breaks your data into clusters and you have regression models for each clusters. Thus, ensuring different sets of data have different models.
For model interpretability, you can use Explain Predictions as @mschmitz suggested, the operator can suggest global variable importance and impact on model base output, as well as, local results of attributes supporting and contradicting the model output for every row. You can also check out SHAP in python, which is a game theory based model explanation package.
Are you trying to use regression clustering together for predictions ? This can achieved by utilizing Hierarchical classification, wherein a clustering algorithm breaks your data into clusters and you have regression models for each clusters. Thus, ensuring different sets of data have different models.
For model interpretability, you can use Explain Predictions as @mschmitz suggested, the operator can suggest global variable importance and impact on model base output, as well as, local results of attributes supporting and contradicting the model output for every row. You can also check out SHAP in python, which is a game theory based model explanation package.