Questions about CUDA and cuDNN versions for Deep learning extensions.
Hi, RapidMiner.
First of all, thank you very much for making such a great operator.
But I have a problem using the Deep learning extension.
The protocol using the deep learning operator of the Deep learning extension works well when the deep learning backend is set to the CPU, but when the deep learning backend is set to the GPU, it rarely uses the GPU. We also found that the computational speed was also slower than when we set backend with the CPU.
My graphics card is GTX 1080 Ti, CUDA version is 9.0.176, cuDNN version is 7.0. We have also set the environment variables for cuDNN.
Have I missed anything? I know you are busy, but I need help. :'-(
Thank you.
Kim.
Find more posts tagged with
Sort by:
1 - 3 of
31
Hi @David_A
Sorry for the late response.
Sorry for the late response.
Here is the nvidia-smi screen when the process is in progress.
And the GPU screen in Task Manager.
The graphics driver version is the minimum version installed automatically when install CUDA 9.0.
The same problem occurs when upgrade this graphics driver to the latest version.
The batch size is 40 and the number of data in the training set is about 4300.
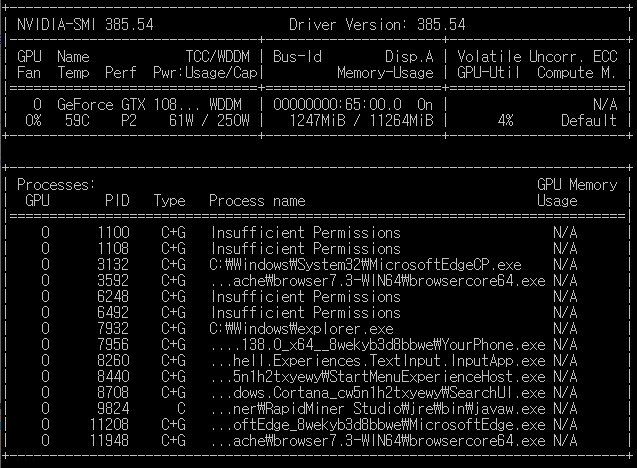
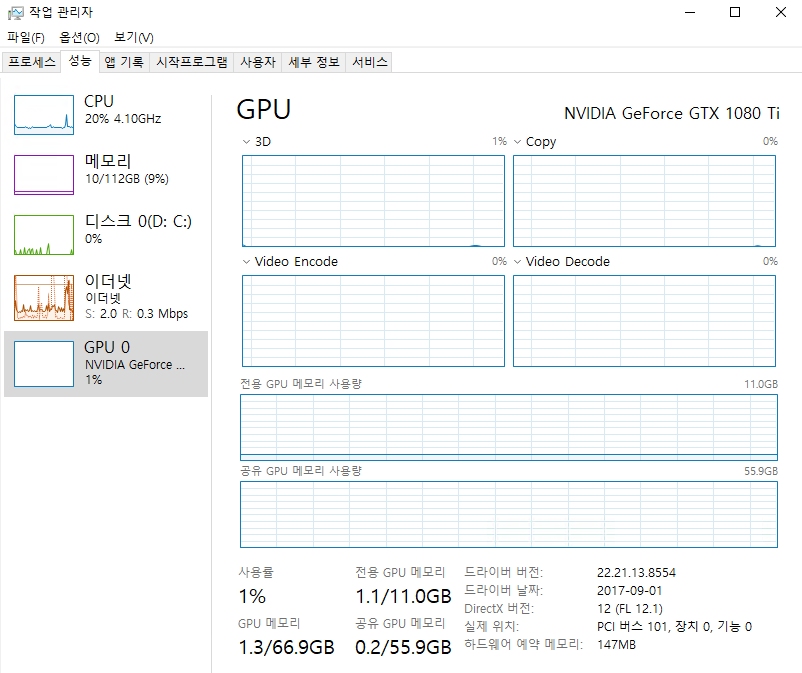
And the GPU screen in Task Manager.
The graphics driver version is the minimum version installed automatically when install CUDA 9.0.
The same problem occurs when upgrade this graphics driver to the latest version.
The batch size is 40 and the number of data in the training set is about 4300.
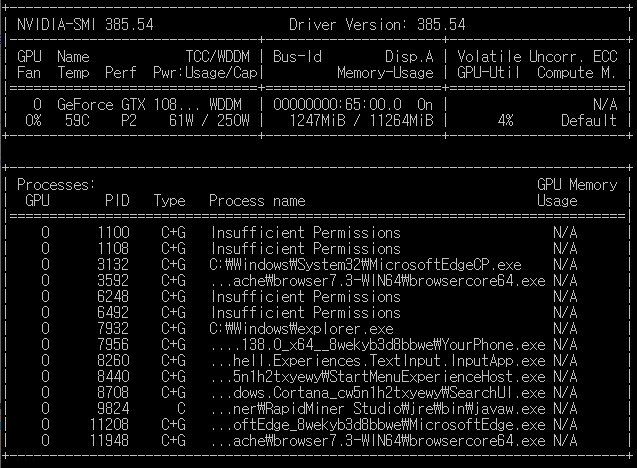
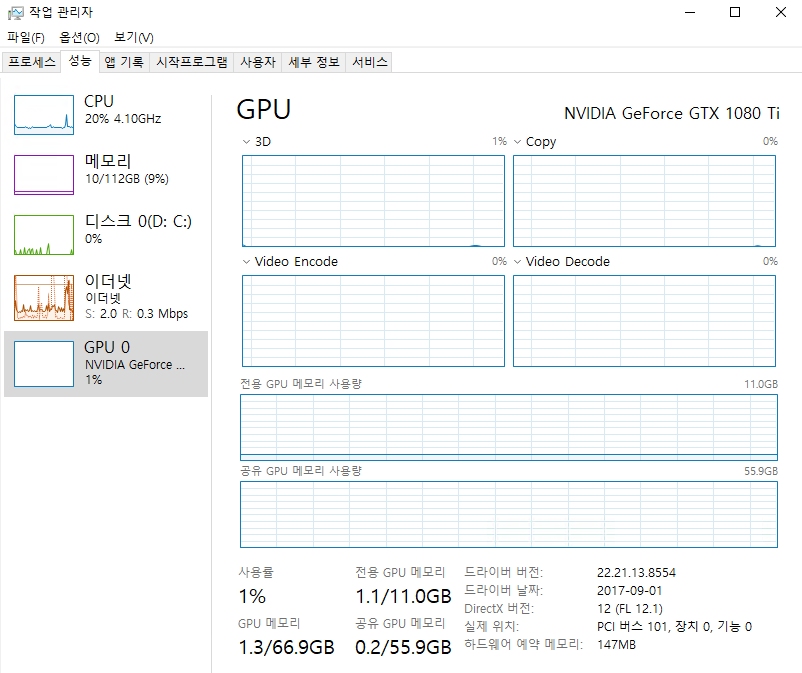
Sort by:
1 - 2 of
21
Hi @KHK ,
your description sounds like everything is set up correctly.
Can you actually successfully switch to GPU back-end in the settings and see actual usage of the GPU when the process is running?
<i>nvidia-smi </i>is a very useful monitoring command to see what actually happens on your GPU.<i><br></i>
One typical issue is, that the (mini-)batch size is too small and therefor only small subsets of the data are actually calculated on the GPU in each iteration. In this case the GPU is done very fast with the calculation and the speed-up is negated by the transfer cost between GPU and the rest of the system.
The same holds true for small data sets and small networks.
Hope that helps a bit,
David
David
Okay, with an example set of this size, the benefit of the GPU is completely negated by the transfer costs of loading the data.
You could try to increase the Batch Size quite a lot (400?), but I assume that you will still have a faster execution by only using the CPU in this case.
<i>nvidia-smi </i>is a very useful monitoring command to see what actually happens on your GPU.<i><br></i>
David