K means Clustering
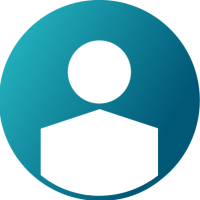
mario_sark
New Altair Community Member
Hello,
I have a quick question, i am build 3 clusters based on RFM Score. R will represent the recent visit to branch , f will represent how often the customer visit within a year , and finally M will represent the amount of money occurs when the customer make a transaction once visit the branch.
once i create the 3 clusters: can re-cluster each cluster into several Clusters based one some variables i choose ?
Thank you
Mario
I have a quick question, i am build 3 clusters based on RFM Score. R will represent the recent visit to branch , f will represent how often the customer visit within a year , and finally M will represent the amount of money occurs when the customer make a transaction once visit the branch.
once i create the 3 clusters: can re-cluster each cluster into several Clusters based one some variables i choose ?
Thank you
Mario
Tagged:
0
Best Answer
-
Or you might not need just 3 clusters to start with. If you have an RFM schema and each dimension has 5 different values, then you have 125 possible combinations. So k-means doesn't need to start with 3 clusters just because you have 3 dimensions--you could set it to however many clusters you think you want, or run X-Means to see what it would recommend.
But as @yyhuang said, if you already have an output target variable in mind, then set it as your label and try a supervised learning algorithm instead. If you want something interpretable, then I have had good results with decision trees and RFM frameworks before.2
Answers
-
Hi @mario_sark,
Are you building something like a hierarchical cluster model?
You can try the top-down clustering operator with flatten. But if you have any ground truth tags in the data, better go supervised.
Your output data will have high-level grouping label and also low-level detailed cluster ID.<?xml version="1.0" encoding="UTF-8"?><process version="9.2.000"> <context> <input/> <output/> <macros/> </context> <operator activated="true" class="process" compatibility="9.2.000" expanded="true" name="Root" origin="GENERATED_TUTORIAL"> <parameter key="logverbosity" value="init"/> <parameter key="random_seed" value="2001"/> <parameter key="send_mail" value="never"/> <parameter key="notification_email" value=""/> <parameter key="process_duration_for_mail" value="30"/> <parameter key="encoding" value="SYSTEM"/> <process expanded="true"> <operator activated="true" class="retrieve" compatibility="9.2.000" expanded="true" height="68" name="Ripley-Set" origin="GENERATED_TUTORIAL" width="90" x="112" y="34"> <parameter key="repository_entry" value="//Samples/data/Ripley-Set"/> </operator> <operator activated="true" class="top_down_clustering" compatibility="9.2.000" expanded="true" height="82" name="Top Down Clustering" origin="GENERATED_TUTORIAL" width="90" x="313" y="238"> <parameter key="create_cluster_label" value="true"/> <parameter key="max_depth" value="5"/> <parameter key="max_leaf_size" value="20"/> <process expanded="true"> <operator activated="true" class="concurrency:k_means" compatibility="9.0.001" expanded="true" height="82" name="K-Means" origin="GENERATED_TUTORIAL" width="90" x="246" y="30"> <parameter key="add_cluster_attribute" value="true"/> <parameter key="add_as_label" value="false"/> <parameter key="remove_unlabeled" value="false"/> <parameter key="k" value="3"/> <parameter key="max_runs" value="10"/> <parameter key="determine_good_start_values" value="false"/> <parameter key="measure_types" value="BregmanDivergences"/> <parameter key="mixed_measure" value="MixedEuclideanDistance"/> <parameter key="nominal_measure" value="NominalDistance"/> <parameter key="numerical_measure" value="EuclideanDistance"/> <parameter key="divergence" value="SquaredEuclideanDistance"/> <parameter key="kernel_type" value="radial"/> <parameter key="kernel_gamma" value="1.0"/> <parameter key="kernel_sigma1" value="1.0"/> <parameter key="kernel_sigma2" value="0.0"/> <parameter key="kernel_sigma3" value="2.0"/> <parameter key="kernel_degree" value="3.0"/> <parameter key="kernel_shift" value="1.0"/> <parameter key="kernel_a" value="1.0"/> <parameter key="kernel_b" value="0.0"/> <parameter key="max_optimization_steps" value="100"/> <parameter key="use_local_random_seed" value="false"/> <parameter key="local_random_seed" value="1992"/> </operator> <connect from_port="example set" to_op="K-Means" to_port="example set"/> <connect from_op="K-Means" from_port="cluster model" to_port="cluster model"/> <portSpacing port="source_example set" spacing="0"/> <portSpacing port="sink_cluster model" spacing="0"/> </process> </operator> <operator activated="true" class="multiply" compatibility="9.2.000" expanded="true" height="103" name="Multiply" width="90" x="514" y="34"/> <operator activated="true" class="flatten_clustering" compatibility="9.2.000" expanded="true" height="82" name="Flatten Clustering" width="90" x="648" y="238"> <parameter key="number_of_clusters" value="3"/> <parameter key="add_as_label" value="true"/> <parameter key="remove_unlabeled" value="false"/> </operator> <connect from_op="Ripley-Set" from_port="output" to_op="Top Down Clustering" to_port="example set"/> <connect from_op="Top Down Clustering" from_port="cluster model" to_op="Multiply" to_port="input"/> <connect from_op="Top Down Clustering" from_port="clustered set" to_op="Flatten Clustering" to_port="example set"/> <connect from_op="Multiply" from_port="output 1" to_port="result 1"/> <connect from_op="Multiply" from_port="output 2" to_op="Flatten Clustering" to_port="hierarchical"/> <connect from_op="Flatten Clustering" from_port="example set" to_port="result 2"/> <portSpacing port="source_input 1" spacing="0"/> <portSpacing port="sink_result 1" spacing="0"/> <portSpacing port="sink_result 2" spacing="0"/> <portSpacing port="sink_result 3" spacing="0"/> </process> </operator> </process>
YY2 -
Or you might not need just 3 clusters to start with. If you have an RFM schema and each dimension has 5 different values, then you have 125 possible combinations. So k-means doesn't need to start with 3 clusters just because you have 3 dimensions--you could set it to however many clusters you think you want, or run X-Means to see what it would recommend.
But as @yyhuang said, if you already have an output target variable in mind, then set it as your label and try a supervised learning algorithm instead. If you want something interpretable, then I have had good results with decision trees and RFM frameworks before.2 -
Hi @yyhuangyyhuang ,
Thank you for you reply ,
these my project Steps:
1- Calculate the RFM
2- Calculate the CP (Customer Power) and give a score
3 - Now i Have as fields : R, F, M, CP
4- Create clusters based on these Variables. (most Prob we want 3 or 4)
5- once we had these clusters we need to do further analysis on each cluster and extract more variables. (maybe 5 variables)
6- now i have more data about my customer in each Cluster. (these that i would use to apply the clustering technique again)
my question was if this is possible to be done. or I have another solution to achieve this Goal
Thank you Again,
Mario
0