How to perform cross-validation correctly when selecting Multi-Objective features?
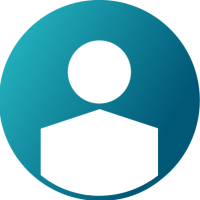
Hello everyone, I am a master student in China and a fan of RapidMiner. However, the time to enter the community is still relatively short and has always been a self-study phase. Thanks to Rapidminer staff, Allie's help, taught me how to post in the RM community. So yes, this is the first time I asked me in such a warm community.
I have recently read a few blog posts, including four articles on multi-objective optimization feature selection and four articles on correct cross-validation (these blog posts are all from Mr. Ingo). I feel inspired. This is worth my further study. My current confusion is that in the fourth blog post on correct cross-validation (https://rapidminer.com/blog/learn-right-way-validate-models-part-4-accidental-contamination/), Ingo Mr. Ms. said that it is to avoid accidentally contaminating data through feature selection. As a result, Ingo conducted cross-validation outside, and internally also had a cross-validation.
In the third multi-objective optimization feature selection blog (https://rapidminer.com/blog/multi-objective-optimization-feature-selection/), Ingo provided a process that directly selects evolutionary features and does not Perform cross-validation. I have been wondering whether it is necessary to conduct cross-validation on the outside in order to achieve the above mentioned correct cross-validation blog mentioned in the avoidance of feature selection to bring about data pollution.
But in multi-objective optimization I do not know how to establish such a process. I want to ask
1. Do you need to add the correct cross-validation step outside? If necessary, how to establish this process? I hope partners and experts help me establish such a correct process. (I have included processes provided by Mr Ingo's blog .It is multi-objective optimization feature selection, and the other is correct cross-validation to avoid accidental contaminationdue to feature selection. How do I merge them?)
2. If there is no need to merge, I also want to listen to the reasons given by my partners and teachers.
Sincerely thanks