Feature selection / reduction text-mining
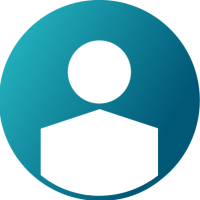
I work with binary unbalanced text-mining classification problem. I have 1000 sentences for one class and 20000 sentences for the outside the class. First I created balance sample (over-sampling/ copy several times). Then I pre-processed the sentences - tokenize, delete stopwords, morphological standardization, filter words less then 2 characters, stem, low case, create n-grams (N=3). I've used TF-IDF for weighting and deleted (prune) n-grams that are rare (occur less then 5% of documents). Then I used C-SVM (LibSVM) (alternative: Bayes) to learn the model from 18000 features. The cross-validation accuracy, recall etc. was great - 98%. Then I used handout set and find that the unseen sentances are classified incorrectly, e.g. sentences that contain informative words from TFIDF list created by the model are classified wrongly. If I use under-sampling the accuracy of my model is only 60%. I am confused.
I presume I have to use some feature reduction/selection techniques (e.g. chi squared, p-value for each n-gram) to improve the situation by I do not understand which to choose how to implement them in RapidMiner or Python. I deleted only 5% of the rare words, but the choice is arbitrary. How can I conduct feature reduction/optimization for text classification in general? What else could cause such problem?