Classification by naive bayes
Dear Community
Good day and Merry Christmas!
I'm still new to this field and try to explore as much knowledge as I can now....
I've a question regarding using naive bayes for classification.
I got a data set with words, sum (in total) and sum (in document), for the further step I wanna use the text mining data to classified the paper which I collected.
However, my process keeps showing ''the example set is empty'', which can be referred to below XML.
Is there any solution for the wrong output? If do, can any expert advise me?
Thanks in advance and wish you all have a nice holiday!
--XML--
Good day and Merry Christmas!
I'm still new to this field and try to explore as much knowledge as I can now....
I've a question regarding using naive bayes for classification.
I got a data set with words, sum (in total) and sum (in document), for the further step I wanna use the text mining data to classified the paper which I collected.
However, my process keeps showing ''the example set is empty'', which can be referred to below XML.
Is there any solution for the wrong output? If do, can any expert advise me?
Thanks in advance and wish you all have a nice holiday!

--XML--
<?xml version="1.0" encoding="UTF-8"?><process version="9.5.001">
<context>
<input/>
<output/>
<macros/>
</context>
<operator activated="true" class="process" compatibility="9.5.001" expanded="true" name="Process">
<parameter key="logverbosity" value="init"/>
<parameter key="random_seed" value="2001"/>
<parameter key="send_mail" value="never"/>
<parameter key="notification_email" value=""/>
<parameter key="process_duration_for_mail" value="30"/>
<parameter key="encoding" value="SYSTEM"/>
<process expanded="true">
<operator activated="true" class="retrieve" compatibility="9.5.001" expanded="true" height="68" name="Retrieve Acceptance 100 rfef result" width="90" x="112" y="85">
<parameter key="repository_entry" value="//Local Repository/new data test/Acceptance 100 rfef result"/>
</operator>
<operator activated="true" class="filter_examples" compatibility="9.5.001" expanded="true" height="103" name="Filter Examples" width="90" x="246" y="85">
<parameter key="parameter_expression" value=""/>
<parameter key="condition_class" value="custom_filters"/>
<parameter key="invert_filter" value="false"/>
<list key="filters_list">
<parameter key="filters_entry_key" value="sum(in documents).ge.75"/>
</list>
<parameter key="filters_logic_and" value="true"/>
<parameter key="filters_check_metadata" value="true"/>
</operator>
<operator activated="true" class="set_role" compatibility="9.5.001" expanded="true" height="82" name="Set Role" width="90" x="380" y="85">
<parameter key="attribute_name" value="word"/>
<parameter key="target_role" value="label"/>
<list key="set_additional_roles"/>
</operator>
<operator activated="true" class="split_validation" compatibility="9.5.001" expanded="true" height="124" name="Validation" width="90" x="514" y="85">
<parameter key="create_complete_model" value="false"/>
<parameter key="split" value="relative"/>
<parameter key="split_ratio" value="0.7"/>
<parameter key="training_set_size" value="100"/>
<parameter key="test_set_size" value="-1"/>
<parameter key="sampling_type" value="automatic"/>
<parameter key="use_local_random_seed" value="false"/>
<parameter key="local_random_seed" value="1992"/>
<process expanded="true">
<operator activated="true" class="naive_bayes" compatibility="9.5.001" expanded="true" height="82" name="Naive Bayes" width="90" x="246" y="34">
<parameter key="laplace_correction" value="true"/>
</operator>
<connect from_port="training" to_op="Naive Bayes" to_port="training set"/>
<connect from_op="Naive Bayes" from_port="model" to_port="model"/>
<portSpacing port="source_training" spacing="0"/>
<portSpacing port="sink_model" spacing="0"/>
<portSpacing port="sink_through 1" spacing="0"/>
</process>
<process expanded="true">
<operator activated="true" class="apply_model" compatibility="9.5.001" expanded="true" height="82" name="Apply Model" width="90" x="112" y="34">
<list key="application_parameters"/>
<parameter key="create_view" value="false"/>
</operator>
<operator activated="true" class="performance_classification" compatibility="9.5.001" expanded="true" height="82" name="Performance" width="90" x="380" y="34">
<parameter key="main_criterion" value="first"/>
<parameter key="accuracy" value="true"/>
<parameter key="classification_error" value="false"/>
<parameter key="kappa" value="false"/>
<parameter key="weighted_mean_recall" value="false"/>
<parameter key="weighted_mean_precision" value="false"/>
<parameter key="spearman_rho" value="false"/>
<parameter key="kendall_tau" value="false"/>
<parameter key="absolute_error" value="false"/>
<parameter key="relative_error" value="false"/>
<parameter key="relative_error_lenient" value="false"/>
<parameter key="relative_error_strict" value="false"/>
<parameter key="normalized_absolute_error" value="false"/>
<parameter key="root_mean_squared_error" value="false"/>
<parameter key="root_relative_squared_error" value="false"/>
<parameter key="squared_error" value="false"/>
<parameter key="correlation" value="false"/>
<parameter key="squared_correlation" value="false"/>
<parameter key="cross-entropy" value="false"/>
<parameter key="margin" value="false"/>
<parameter key="soft_margin_loss" value="false"/>
<parameter key="logistic_loss" value="false"/>
<parameter key="skip_undefined_labels" value="true"/>
<parameter key="use_example_weights" value="true"/>
<list key="class_weights"/>
</operator>
<connect from_port="model" to_op="Apply Model" to_port="model"/>
<connect from_port="test set" to_op="Apply Model" to_port="unlabelled data"/>
<connect from_op="Apply Model" from_port="labelled data" to_op="Performance" to_port="labelled data"/>
<connect from_op="Performance" from_port="performance" to_port="averagable 1"/>
<portSpacing port="source_model" spacing="0"/>
<portSpacing port="source_test set" spacing="0"/>
<portSpacing port="source_through 1" spacing="0"/>
<portSpacing port="sink_averagable 1" spacing="0"/>
<portSpacing port="sink_averagable 2" spacing="0"/>
</process>
</operator>
<connect from_op="Retrieve Acceptance 100 rfef result" from_port="output" to_op="Filter Examples" to_port="example set input"/>
<connect from_op="Filter Examples" from_port="example set output" to_op="Set Role" to_port="example set input"/>
<connect from_op="Set Role" from_port="example set output" to_op="Validation" to_port="training"/>
<connect from_op="Validation" from_port="model" to_port="result 1"/>
<connect from_op="Validation" from_port="training" to_port="result 2"/>
<connect from_op="Validation" from_port="averagable 1" to_port="result 3"/>
<portSpacing port="source_input 1" spacing="0"/>
<portSpacing port="sink_result 1" spacing="0"/>
<portSpacing port="sink_result 2" spacing="0"/>
<portSpacing port="sink_result 3" spacing="0"/>
<portSpacing port="sink_result 4" spacing="0"/>
</process>
</operator>
</process>
Find more posts tagged with
Sort by:
1 - 5 of
51
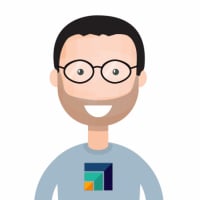
hi @Ka13n can you also post your repository data set "Acceptance 100 rfef result"? We cannot run your process and replicate your result without it.
Scott