Gradient Boosted Tree Algorithm performance
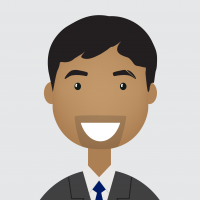
varunm1
New Altair Community Member
I am working with Gradient boosted tree (GBT), and it performs better (5-Fold CV) on most of my datasets with high metrics like AUC (1.0), kappa (0.971), etc. I can correlate the results with the capabilities of GBT like regularization and sequential learning. I even set aside 30 percent data for testing after five-fold cross-validation and got kappa (0.974) for this unseen data.
My question is, are there any cautions or factors that need to be considered while using and interpreting results of a GBT and how good is GBT in real applications?
Thanks
My question is, are there any cautions or factors that need to be considered while using and interpreting results of a GBT and how good is GBT in real applications?
Thanks
Tagged:
0
Best Answers
-
GBTs are great in terms of predictions. In terms of interpretability, I think they are somewhat harder because the trees are boosted and not independent (so less interpretable than an Random Forest, in my view). But as long as you are using other ways to communicate model results (including some of the great tools in RapidMiner like simulation and explaining predictions) then they are fine.
You did mention AUC of 1.0 and that is pretty much perfect separation, so also make sure that you don't have any data leakage or sample contamination going on. Nothing is worse than deploying a model in production and watching its performance collapse!5 -
@varunm1 ,sorry i am a bit busy. But to clarify: Are you sure that each ID is really independed from the other? These are really different customers or different machines etc? These are NOT correlated examples like the same customer in different years or an item generated in the same batch than others?Best,Martin5
Answers
-
Hi @varunm1 ,well, the usual overtraining thing for any complex algorithm, which you are already consindering. Usually GBTs are the best off-the-shelf algorithm, especially if you have nominal data in your data set.BR,Martin1
-
GBTs are great in terms of predictions. In terms of interpretability, I think they are somewhat harder because the trees are boosted and not independent (so less interpretable than an Random Forest, in my view). But as long as you are using other ways to communicate model results (including some of the great tools in RapidMiner like simulation and explaining predictions) then they are fine.
You did mention AUC of 1.0 and that is pretty much perfect separation, so also make sure that you don't have any data leakage or sample contamination going on. Nothing is worse than deploying a model in production and watching its performance collapse!5 -
Thanks, @Telcontar120. I am a bit skeptic about the result so, I did some basic checks like the correlation of attributes and hold out dataset (30%) for testing after CV and test set looks good as well. Will check other methods as well to see if there are any issues.
0 -
Are you sure that your hold out is really independed and not pseudo-duplicates?Best,Martin0
-
@varunm1 ,sorry i am a bit busy. But to clarify: Are you sure that each ID is really independed from the other? These are really different customers or different machines etc? These are NOT correlated examples like the same customer in different years or an item generated in the same batch than others?Best,Martin5
-
yes, they are different subjects. I tested by setting some subject aside for testing and applied cross-validation on remaining data. Cross-validation result looks pretty good but the test results on the separated subjects are poor.
0 -
@mschmitz thanks. I have gone through your experience posted in the below link and it suits this scenario as well. @lionelderkrikor thanks for solving the filter operator issue.
https://towardsdatascience.com/when-cross-validation-fails-9bd5a57f07b5
1 -
Is there a way to do Subject wise cross-validation in RM rather than the record-wise (default) cross-validation. Subject wise cross-validation is where we split data based on Subject ID column rather than randomly splitting data into different folds. Leave one out subject wise cross-validation is recommended in case of medical diagnosis.
This is especially used when there are multiple samples per subject in the dataset.
Thanks a lot for your support.0 -
Hi,There used to be an operator called "Batch Validation" which could have been used for that, but it seems like this one was removed in version 7.3 when we introduced parallel processing for all the validation operators. With this operator you would have specified a "batch" attribute which defined the splits for the cross validation. In your case this would have been the subject IDs (or more likely: groups of subjects).Anyway, since this operator is history, below is a simple process to achieve the same. I use the passenger class of Titanic to define the groups. In your case, you would use groups containing the same subject(s).If there is a lot of calling for such an operator in the future, I am sure we can bring it back but for now this should be a good workaround...Hope this helps,
IngoSpoiler<?xml version="1.0" encoding="UTF-8"?><process version="9.2.000"><br> <context><br> <input/><br> <output/><br> <macros/><br> </context><br> <operator activated="true" class="process" compatibility="9.2.000" expanded="true" name="Process"><br> <parameter key="logverbosity" value="init"/><br> <parameter key="random_seed" value="2001"/><br> <parameter key="send_mail" value="never"/><br> <parameter key="notification_email" value=""/><br> <parameter key="process_duration_for_mail" value="30"/><br> <parameter key="encoding" value="UTF-8"/><br> <process expanded="true"><br> <operator activated="true" class="retrieve" compatibility="9.2.000" expanded="true" height="68" name="Retrieve Titanic Training" width="90" x="45" y="34"><br> <parameter key="repository_entry" value="//Samples/data/Titanic Training"/><br> </operator><br> <operator activated="true" class="set_role" compatibility="9.2.000" expanded="true" height="82" name="Set Role" width="90" x="179" y="34"><br> <parameter key="attribute_name" value="Survived"/><br> <parameter key="target_role" value="label"/><br> <list key="set_additional_roles"/><br> </operator><br> <operator activated="true" class="concurrency:loop_values" compatibility="9.2.000" expanded="true" height="82" name="Loop Values" width="90" x="313" y="34"><br> <parameter key="attribute" value="Passenger Class"/><br> <parameter key="iteration_macro" value="loop_value"/><br> <parameter key="reuse_results" value="false"/><br> <parameter key="enable_parallel_execution" value="true"/><br> <process expanded="true"><br> <operator activated="true" class="filter_examples" compatibility="9.2.000" expanded="true" height="103" name="Filter Examples" width="90" x="45" y="85"><br> <parameter key="parameter_expression" value=""/><br> <parameter key="condition_class" value="custom_filters"/><br> <parameter key="invert_filter" value="false"/><br> <list key="filters_list"><br> <parameter key="filters_entry_key" value="Passenger Class.equals.%{loop_value}"/><br> </list><br> <parameter key="filters_logic_and" value="true"/><br> <parameter key="filters_check_metadata" value="true"/><br> </operator><br> <operator activated="true" class="select_attributes" compatibility="9.2.000" expanded="true" height="82" name="Select Attributes (2)" width="90" x="179" y="136"><br> <parameter key="attribute_filter_type" value="single"/><br> <parameter key="attribute" value="Passenger Class"/><br> <parameter key="attributes" value=""/><br> <parameter key="use_except_expression" value="false"/><br> <parameter key="value_type" value="attribute_value"/><br> <parameter key="use_value_type_exception" value="false"/><br> <parameter key="except_value_type" value="time"/><br> <parameter key="block_type" value="attribute_block"/><br> <parameter key="use_block_type_exception" value="false"/><br> <parameter key="except_block_type" value="value_matrix_row_start"/><br> <parameter key="invert_selection" value="true"/><br> <parameter key="include_special_attributes" value="false"/><br> </operator><br> <operator activated="true" class="concurrency:parallel_decision_tree" compatibility="9.2.000" expanded="true" height="103" name="Decision Tree" width="90" x="313" y="136"><br> <parameter key="criterion" value="gain_ratio"/><br> <parameter key="maximal_depth" value="10"/><br> <parameter key="apply_pruning" value="true"/><br> <parameter key="confidence" value="0.1"/><br> <parameter key="apply_prepruning" value="true"/><br> <parameter key="minimal_gain" value="0.01"/><br> <parameter key="minimal_leaf_size" value="2"/><br> <parameter key="minimal_size_for_split" value="4"/><br> <parameter key="number_of_prepruning_alternatives" value="3"/><br> </operator><br> <operator activated="true" class="select_attributes" compatibility="9.2.000" expanded="true" height="82" name="Select Attributes" width="90" x="179" y="34"><br> <parameter key="attribute_filter_type" value="single"/><br> <parameter key="attribute" value="Passenger Class"/><br> <parameter key="attributes" value=""/><br> <parameter key="use_except_expression" value="false"/><br> <parameter key="value_type" value="attribute_value"/><br> <parameter key="use_value_type_exception" value="false"/><br> <parameter key="except_value_type" value="time"/><br> <parameter key="block_type" value="attribute_block"/><br> <parameter key="use_block_type_exception" value="false"/><br> <parameter key="except_block_type" value="value_matrix_row_start"/><br> <parameter key="invert_selection" value="true"/><br> <parameter key="include_special_attributes" value="false"/><br> </operator><br> <operator activated="true" class="apply_model" compatibility="9.2.000" expanded="true" height="82" name="Apply Model" width="90" x="447" y="34"><br> <list key="application_parameters"/><br> <parameter key="create_view" value="false"/><br> </operator><br> <operator activated="true" class="performance_classification" compatibility="9.2.000" expanded="true" height="82" name="Performance" width="90" x="581" y="34"><br> <parameter key="main_criterion" value="first"/><br> <parameter key="accuracy" value="true"/><br> <parameter key="classification_error" value="false"/><br> <parameter key="kappa" value="false"/><br> <parameter key="weighted_mean_recall" value="false"/><br> <parameter key="weighted_mean_precision" value="false"/><br> <parameter key="spearman_rho" value="false"/><br> <parameter key="kendall_tau" value="false"/><br> <parameter key="absolute_error" value="false"/><br> <parameter key="relative_error" value="false"/><br> <parameter key="relative_error_lenient" value="false"/><br> <parameter key="relative_error_strict" value="false"/><br> <parameter key="normalized_absolute_error" value="false"/><br> <parameter key="root_mean_squared_error" value="false"/><br> <parameter key="root_relative_squared_error" value="false"/><br> <parameter key="squared_error" value="false"/><br> <parameter key="correlation" value="false"/><br> <parameter key="squared_correlation" value="false"/><br> <parameter key="cross-entropy" value="false"/><br> <parameter key="margin" value="false"/><br> <parameter key="soft_margin_loss" value="false"/><br> <parameter key="logistic_loss" value="false"/><br> <parameter key="skip_undefined_labels" value="true"/><br> <parameter key="use_example_weights" value="true"/><br> <list key="class_weights"/><br> </operator><br> <connect from_port="input 1" to_op="Filter Examples" to_port="example set input"/><br> <connect from_op="Filter Examples" from_port="example set output" to_op="Select Attributes" to_port="example set input"/><br> <connect from_op="Filter Examples" from_port="unmatched example set" to_op="Select Attributes (2)" to_port="example set input"/><br> <connect from_op="Select Attributes (2)" from_port="example set output" to_op="Decision Tree" to_port="training set"/><br> <connect from_op="Decision Tree" from_port="model" to_op="Apply Model" to_port="model"/><br> <connect from_op="Select Attributes" from_port="example set output" to_op="Apply Model" to_port="unlabelled data"/><br> <connect from_op="Apply Model" from_port="labelled data" to_op="Performance" to_port="labelled data"/><br> <connect from_op="Performance" from_port="performance" to_port="output 1"/><br> <portSpacing port="source_input 1" spacing="0"/><br> <portSpacing port="source_input 2" spacing="0"/><br> <portSpacing port="sink_output 1" spacing="0"/><br> <portSpacing port="sink_output 2" spacing="0"/><br> </process><br> </operator><br> <operator activated="true" class="average" compatibility="9.2.000" expanded="true" height="82" name="Average" width="90" x="447" y="34"/><br> <connect from_op="Retrieve Titanic Training" from_port="output" to_op="Set Role" to_port="example set input"/><br> <connect from_op="Set Role" from_port="example set output" to_op="Loop Values" to_port="input 1"/><br> <connect from_op="Loop Values" from_port="output 1" to_op="Average" to_port="averagable 1"/><br> <connect from_op="Average" from_port="average" to_port="result 1"/><br> <portSpacing port="source_input 1" spacing="0"/><br> <portSpacing port="sink_result 1" spacing="0"/><br> <portSpacing port="sink_result 2" spacing="0"/><br> </process><br> </operator><br></process>
1 -
Doesn't the standard cross-validation still have the "split on batch attribute" available as an advanced parameter? Is that not doing this same thing?1
-
Hello @Telcontar120
Thanks for your response, looks like it is doing the same. I tested the CV with a split on batch attribute, the performance metrics are the same as process provided by Ingo. Any suggestion on doing similar cv with different folds (5 or 10) rather than testing on an individual batch. This is because once I select the CV with "split on batch attribute" the option for the number of folds disappears.0 -
Right, once you are using your own batches, you will have as many folds as you have unique values of your batch attribute. I am not entirely sure what you mean by doing multiple folds while also using a batch that specifies the records to be used in each fold. If you mean you have a set number of batches and you want cross-validation performed on each batch (rather than just one model on each batch) you could simply put a conventional cross-validation inside the testing side of your outer cross-validation with which you are splitting on batch. That should do the trick.0
-
Thank you @Telcontar120 I have an ID column which has 87 unique id values (1500 samples). Now I want to perform 5 fold cross-validation based on ID values rather than samples. If I select split on the batch attribute and set ID column role as batch it does "leave one subject out cross-validation" in my case. But if I want to perform 5 fold CV based on ID ( samples related to 60 IDs in train and 17 in the test), I don't see there is an option for this in the CV operator.
Sorry if it is confusing.0 -
Hi @varunm1
you can go for Generate Attribute with
batchid = id%5
then use Set Role to make this the role "batch" and use the batch option of x-val.
BR,
Martin2