How can we see the threshold chosen by the auto model classification model for final confusion mtx
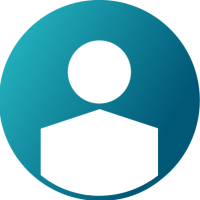
unm
New Altair Community Member
The auto model we created uses GBTree and produces a confusion matrix. We would like to see what threshold it had used for creating this matrix. Is there a way to view the threshold used?
Tagged:
0
Best Answers
-
Hi @unm
(If I am mistaken, let the gurus correct me)
In general, threshold choice is a separate problem to be solved and is dependant on many factors, but in real life - mostly business metrics.
Most binary classifiers are capable of producing two types of predictions, one is 1/0 (True/False, Yes/No) and the other is a probability of a certain class. By default, the threshold is always in the middle - which means, "< 0.5 = class1" and "> 0.5 = class2". This is how any confusion matrix in RapidMiner is built (this includes Auto Model as well), in case you didn't explicitly used in your process, for example, SET THRESHOLD and APPLY THRESHOLD operators, in order to move the threshold in a desired direction (higher / lower).
2 -
You can find/verify the threshold by sorting the prediction score and seeing at which value the switch in prediction occurs. It is almost certain that the algorithm is simply using the default of 0.50 but as @kypexin says you can also modify that with additional operators in RapidMiner.1
Answers
-
Hi @unm
(If I am mistaken, let the gurus correct me)
In general, threshold choice is a separate problem to be solved and is dependant on many factors, but in real life - mostly business metrics.
Most binary classifiers are capable of producing two types of predictions, one is 1/0 (True/False, Yes/No) and the other is a probability of a certain class. By default, the threshold is always in the middle - which means, "< 0.5 = class1" and "> 0.5 = class2". This is how any confusion matrix in RapidMiner is built (this includes Auto Model as well), in case you didn't explicitly used in your process, for example, SET THRESHOLD and APPLY THRESHOLD operators, in order to move the threshold in a desired direction (higher / lower).
2 -
You can find/verify the threshold by sorting the prediction score and seeing at which value the switch in prediction occurs. It is almost certain that the algorithm is simply using the default of 0.50 but as @kypexin says you can also modify that with additional operators in RapidMiner.1
-
Thanks @kypexin and @Telcontar120. Really appreciate your time answering this. Yes, we guessed so (As 0.5 as the threshold) but wanted to confirm it to see if its doing anything more intelligently. That answers the question!1
-
Hi,We actually have been discussing this a bit. It is hard to do this in a really intelligent way for the reasons @kypexin has been mentioning. Without knowing the business context, one value is almost as good as any other :-)However, there are three ways from here to potentially improve this a bit:
- Offer a full-blown cost matrix based approach for Auto Model and perform a threshold optimization for optimizing profits / costs
- Optimize thresholds in a way that Accuracy (or F-Measure or...) is maximized
- Do nothing and leave it as it is
I personally do not like No 1 since it would take away some of the simplicity of AM in the early prototyping phase. But I see the benefits of course and could imagine to make this optional.No 2 is at least avoiding problems with strongly imbalanced data sets and is what many internal people here at RM would love to see for AM.No 3 is very efficient in terms of resourcesI appreciate any opinion here (including additional ideas). We may be able to improve this for one of the future releases if we have a good plan which is widely preferred.Thanks,Ingo1 -
Personally I think option #3 has the virtue of simplicity as well as efficiency---and thus is a good choice for automodel. Many users of automodel might not understand the nuances of threshhold selection and modification and I fear that if you incorporate that automatically into automodel (such as option #2) then that could lead to additional confusion and misunderstanding later. So my vote would be to keep option #3.
2