How to plot train accuracy vs. test accuracy?
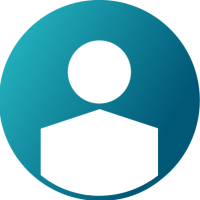
hi,
currently I am testing some configuration parameters C and gamma with a SVM inside X-Val inside Opt.Parameter Operator...
the process looks like this:
<?xml version="1.0" encoding="UTF-8"?><process version="7.2.000">
<context>
<input/>
<output/>
<macros/>
</context>
<operator activated="true" class="process" compatibility="7.2.000" expanded="true" name="Process">
<process expanded="true">
<operator activated="false" class="retrieve" compatibility="7.2.000" expanded="true" height="68" name="Retrieve" width="90" x="45" y="238">
<parameter key="repository_entry" value="//RapidMiner_Nils/Nils/Master/Data/Master Excelliste_Gefügebezeichnung_3 klassen"/>
</operator>
<operator activated="false" class="split_data" compatibility="7.2.000" expanded="true" height="103" name="Split Data" width="90" x="179" y="238">
<enumeration key="partitions">
<parameter key="ratio" value="0.5"/>
<parameter key="ratio" value="0.5"/>
</enumeration>
<parameter key="sampling_type" value="stratified sampling"/>
<parameter key="use_local_random_seed" value="true"/>
</operator>
<operator activated="false" class="write_excel" compatibility="7.2.000" expanded="true" height="82" name="Write Excel (2)" width="90" x="45" y="442">
<parameter key="excel_file" value="C:\Users\Admin\Desktop\testData.xlsx"/>
</operator>
<operator activated="false" class="write_excel" compatibility="7.2.000" expanded="true" height="82" name="Write Excel" width="90" x="45" y="136">
<parameter key="excel_file" value="C:\Users\Admin\Desktop\trainData.xlsx"/>
</operator>
<operator activated="true" class="retrieve" compatibility="7.2.000" expanded="true" height="68" name="Retrieve testData" width="90" x="179" y="391">
<parameter key="repository_entry" value="//RapidMiner_Nils/repositories/Local Repository/data/test und training/testData"/>
</operator>
<operator activated="true" class="normalize" compatibility="7.2.000" expanded="true" height="103" name="Normalize Test Data" width="90" x="313" y="391"/>
<operator activated="true" class="retrieve" compatibility="7.2.000" expanded="true" height="68" name="Retrieve trainData" width="90" x="179" y="136">
<parameter key="repository_entry" value="//RapidMiner_Nils/repositories/Local Repository/data/test und training/trainData"/>
</operator>
<operator activated="true" class="normalize" compatibility="7.2.000" expanded="true" height="103" name="Normalize" width="90" x="45" y="34"/>
<operator activated="true" class="log" compatibility="7.2.000" expanded="true" height="82" name="Log Normaize Parameter" width="90" x="179" y="34">
<list key="log">
<parameter key="attributes" value="operator.Normalize.parameter.attributes"/>
<parameter key="value type" value="operator.Normalize.parameter.value_type"/>
</list>
</operator>
<operator activated="true" class="multiply" compatibility="7.2.000" expanded="true" height="124" name="Multiply Trainings Data" width="90" x="313" y="34"/>
<operator activated="true" class="optimize_parameters_grid" compatibility="7.2.000" expanded="true" height="103" name="Optimize Parameters (Grid)" width="90" x="648" y="34">
<list key="parameters">
<parameter key="SVM.C" value="[1000;300000;10;linear]"/>
<parameter key="SVM.gamma" value="[0.001;1;10;linear]"/>
</list>
<process expanded="true">
<operator activated="true" class="x_validation" compatibility="7.2.000" expanded="true" height="124" name="Validation" width="90" x="313" y="34">
<parameter key="number_of_validations" value="5"/>
<process expanded="true">
<operator activated="true" class="support_vector_machine_libsvm" compatibility="7.2.000" expanded="true" height="82" name="SVM" width="90" x="246" y="34">
<parameter key="gamma" value="1.0"/>
<parameter key="C" value="300000.0"/>
<list key="class_weights"/>
</operator>
<connect from_port="training" to_op="SVM" to_port="training set"/>
<connect from_op="SVM" from_port="model" to_port="model"/>
<portSpacing port="source_training" spacing="0"/>
<portSpacing port="sink_model" spacing="0"/>
<portSpacing port="sink_through 1" spacing="0"/>
</process>
<process expanded="true">
<operator activated="true" class="apply_model" compatibility="7.2.000" expanded="true" height="82" name="Apply Model" width="90" x="112" y="34">
<list key="application_parameters"/>
</operator>
<operator activated="true" class="performance_classification" compatibility="7.2.000" expanded="true" height="82" name="Performance" width="90" x="313" y="34">
<list key="class_weights"/>
</operator>
<connect from_port="model" to_op="Apply Model" to_port="model"/>
<connect from_port="test set" to_op="Apply Model" to_port="unlabelled data"/>
<connect from_op="Apply Model" from_port="labelled data" to_op="Performance" to_port="labelled data"/>
<connect from_op="Performance" from_port="performance" to_port="averagable 1"/>
<portSpacing port="source_model" spacing="0"/>
<portSpacing port="source_test set" spacing="0"/>
<portSpacing port="source_through 1" spacing="0"/>
<portSpacing port="sink_averagable 1" spacing="0"/>
<portSpacing port="sink_averagable 2" spacing="0"/>
</process>
</operator>
<operator activated="true" class="log" compatibility="7.2.000" expanded="true" height="82" name="Log" width="90" x="648" y="85">
<list key="log">
<parameter key="C" value="operator.SVM.parameter.C"/>
<parameter key="gamma" value="operator.SVM.parameter.gamma"/>
<parameter key="XVAL_Performance" value="operator.Validation.value.performance"/>
<parameter key="XVAL_iteration" value="operator.Validation.value.iteration"/>
<parameter key="XVAL_time" value="operator.Validation.value.time"/>
<parameter key="Perf_acc" value="operator.Performance.value.accuracy"/>
<parameter key="Perf_kappa" value="operator.Performance.value.kappa"/>
<parameter key="Perf_time" value="operator.Performance.value.cpu-time"/>
<parameter key="TESTPERF_acc" value="operator.TESTPERF.value.accuracy"/>
<parameter key="TESTPERF_kappa" value="operator.TESTPERF.value.kappa"/>
<parameter key="TRAINPERF_acc" value="operator.TRAINPERF.value.accuracy"/>
<parameter key="TRAINPERF_kappa" value="operator.TRAINPERF.value.kappa"/>
<parameter key="TESTPERF_time" value="operator.TESTPERF.value.time"/>
<parameter key="TRAINPERF_time" value="operator.TRAINPERF.value.time"/>
</list>
</operator>
<connect from_port="input 1" to_op="Validation" to_port="training"/>
<connect from_op="Validation" from_port="averagable 1" to_op="Log" to_port="through 1"/>
<connect from_op="Log" from_port="through 1" to_port="performance"/>
<portSpacing port="source_input 1" spacing="0"/>
<portSpacing port="source_input 2" spacing="0"/>
<portSpacing port="sink_performance" spacing="0"/>
<portSpacing port="sink_result 1" spacing="0"/>
</process>
</operator>
<operator activated="true" class="set_parameters" compatibility="7.2.000" expanded="true" height="82" name="Set Parameters" width="90" x="849" y="85">
<list key="name_map">
<parameter key="SVM" value="SVM Trainings Data"/>
</list>
</operator>
<operator activated="true" class="support_vector_machine_libsvm" compatibility="7.2.000" expanded="true" height="82" name="SVM Trainings Data" width="90" x="581" y="187">
<parameter key="gamma" value="0.001"/>
<parameter key="C" value="270100.0"/>
<list key="class_weights"/>
</operator>
<operator activated="true" class="multiply" compatibility="7.2.000" expanded="true" height="103" name="Multiply Model" width="90" x="782" y="187"/>
<operator activated="true" class="apply_model" compatibility="7.2.000" expanded="true" height="82" name="Apply Model (2)" width="90" x="447" y="289">
<list key="application_parameters"/>
</operator>
<operator activated="true" class="performance_classification" compatibility="7.2.000" expanded="true" height="82" name="TRAINPERF" width="90" x="715" y="340">
<parameter key="classification_error" value="true"/>
<list key="class_weights"/>
</operator>
<operator activated="true" class="apply_model" compatibility="7.2.000" expanded="true" height="82" name="Apply Model (3)" width="90" x="581" y="442">
<list key="application_parameters"/>
</operator>
<operator activated="true" class="performance_classification" compatibility="7.2.000" expanded="true" height="82" name="TESTPERF" width="90" x="715" y="442">
<parameter key="classification_error" value="true"/>
<list key="class_weights"/>
</operator>
<operator activated="true" class="log" compatibility="7.2.000" expanded="true" height="103" name="LOG ALL" width="90" x="849" y="340">
<list key="log">
<parameter key="accuracy" value="operator.Performance.value.accuracy"/>
<parameter key="classification error" value="operator.Performance.value.classification_error"/>
</list>
</operator>
<connect from_op="Retrieve" from_port="output" to_op="Split Data" to_port="example set"/>
<connect from_op="Split Data" from_port="partition 1" to_op="Write Excel" to_port="input"/>
<connect from_op="Split Data" from_port="partition 2" to_op="Write Excel (2)" to_port="input"/>
<connect from_op="Retrieve testData" from_port="output" to_op="Normalize Test Data" to_port="example set input"/>
<connect from_op="Normalize Test Data" from_port="example set output" to_op="Apply Model (3)" to_port="unlabelled data"/>
<connect from_op="Retrieve trainData" from_port="output" to_op="Normalize" to_port="example set input"/>
<connect from_op="Normalize" from_port="example set output" to_op="Log Normaize Parameter" to_port="through 1"/>
<connect from_op="Log Normaize Parameter" from_port="through 1" to_op="Multiply Trainings Data" to_port="input"/>
<connect from_op="Multiply Trainings Data" from_port="output 1" to_op="SVM Trainings Data" to_port="training set"/>
<connect from_op="Multiply Trainings Data" from_port="output 2" to_op="Apply Model (2)" to_port="unlabelled data"/>
<connect from_op="Multiply Trainings Data" from_port="output 3" to_op="Optimize Parameters (Grid)" to_port="input 1"/>
<connect from_op="Optimize Parameters (Grid)" from_port="performance" to_port="result 1"/>
<connect from_op="Optimize Parameters (Grid)" from_port="parameter" to_op="Set Parameters" to_port="parameter set"/>
<connect from_op="Set Parameters" from_port="parameter set" to_port="result 3"/>
<connect from_op="SVM Trainings Data" from_port="model" to_op="Multiply Model" to_port="input"/>
<connect from_op="Multiply Model" from_port="output 1" to_op="Apply Model (2)" to_port="model"/>
<connect from_op="Multiply Model" from_port="output 2" to_op="Apply Model (3)" to_port="model"/>
<connect from_op="Apply Model (2)" from_port="labelled data" to_op="TRAINPERF" to_port="labelled data"/>
<connect from_op="TRAINPERF" from_port="performance" to_op="LOG ALL" to_port="through 1"/>
<connect from_op="Apply Model (3)" from_port="labelled data" to_op="TESTPERF" to_port="labelled data"/>
<connect from_op="TESTPERF" from_port="performance" to_op="LOG ALL" to_port="through 2"/>
<connect from_op="LOG ALL" from_port="through 1" to_port="result 2"/>
<portSpacing port="source_input 1" spacing="0"/>
<portSpacing port="sink_result 1" spacing="0"/>
<portSpacing port="sink_result 2" spacing="0"/>
<portSpacing port="sink_result 3" spacing="0"/>
<portSpacing port="sink_result 4" spacing="0"/>
</process>
</operator>
</process>
what I would really like to achieve, is going to the different C and gamma with their respective performance, BOTH FOR TESTING AND TRAINING VALIDATION (+performance) and plot them together with C, gamma and their performance in a single graph.. best would be a series graph maybe...
the thing is, I want to see when OVERFITTING occurs, like see a discrepancy some time when training accuracy goes up, and when (on what C and gamma configuration) the testing performance decreases ...
if I could also plot X-Val performance together with the others in the graph, that would be perfect..
is this somehow realisable in Rapidminer? maybe in series multiple, but I have the problem, that in my current configuration I get only best parameters C and gamma to be set for the testing and training model, how can I apply C and gamma for all possible grid combinations and set them for the test/ training run, so that at each configuration, also the test/train validation+performance is executed?
Answers
-
Hi,
I am afraid that I can't build this right into your process this time but at least I build a process showing you how to calculate this in general - I am sure you can apply those concepts to your process.
Here is a screenshot of the result:
As you can see, somewhere around a value of 1.2 to 1.3 for C, the SVM begins to overfit. The training error (red) is still reduced while the testing error (blue) starts to grow again. But here is the thing: I needed roughly 30 minutes of data set generation and parameter range tuning to actually GET to this point. SVMs are in general pretty good at avoiding overfitting and if I would have the blue curve alone and minimize for the test value, I would end up with exactly the same value. So showing the red curve at all does not really help me.
But, hey, you know my opinion of training error by now so don't expect me to be excited about this :smileywink:
Here is the process:
<?xml version="1.0" encoding="UTF-8"?><process version="7.2.000">
<context>
<input/>
<output/>
<macros/>
</context>
<operator activated="true" class="process" compatibility="7.2.000" expanded="true" name="Root">
<process expanded="true">
<operator activated="true" class="generate_data" compatibility="7.2.000" expanded="true" height="68" name="Generate Data" width="90" x="45" y="34">
<parameter key="target_function" value="sum"/>
</operator>
<operator activated="true" class="add_noise" compatibility="7.2.000" expanded="true" height="103" name="Add Noise" width="90" x="179" y="34">
<parameter key="random_attributes" value="5"/>
<parameter key="label_noise" value="0.5"/>
<list key="noise"/>
</operator>
<operator activated="true" class="optimize_parameters_grid" compatibility="7.2.000" expanded="true" height="103" name="ParameterOptimization" width="90" x="313" y="34">
<list key="parameters">
<parameter key="Training.C" value="[0.01;3;40;linear]"/>
</list>
<process expanded="true">
<operator activated="true" class="x_validation" compatibility="7.2.000" expanded="true" height="124" name="Validation" width="90" x="45" y="34">
<parameter key="sampling_type" value="shuffled sampling"/>
<parameter key="use_local_random_seed" value="true"/>
<process expanded="true">
<operator activated="true" class="support_vector_machine_libsvm" compatibility="7.2.000" expanded="true" height="82" name="Training" width="90" x="112" y="34">
<parameter key="svm_type" value="epsilon-SVR"/>
<parameter key="degree" value="10"/>
<parameter key="C" value="3.0"/>
<parameter key="epsilon" value="0.01"/>
<list key="class_weights"/>
</operator>
<connect from_port="training" to_op="Training" to_port="training set"/>
<connect from_op="Training" from_port="model" to_port="model"/>
<portSpacing port="source_training" spacing="0"/>
<portSpacing port="sink_model" spacing="0"/>
<portSpacing port="sink_through 1" spacing="0"/>
</process>
<process expanded="true">
<operator activated="true" class="apply_model" compatibility="7.1.001" expanded="true" height="82" name="Test" width="90" x="112" y="34">
<list key="application_parameters"/>
</operator>
<operator activated="true" class="performance_regression" compatibility="7.2.000" expanded="true" height="82" name="Evaluation" width="90" x="313" y="34">
<parameter key="root_mean_squared_error" value="false"/>
<parameter key="absolute_error" value="true"/>
<parameter key="normalized_absolute_error" value="true"/>
<parameter key="squared_error" value="true"/>
</operator>
<connect from_port="model" to_op="Test" to_port="model"/>
<connect from_port="test set" to_op="Test" to_port="unlabelled data"/>
<connect from_op="Test" from_port="labelled data" to_op="Evaluation" to_port="labelled data"/>
<connect from_op="Evaluation" from_port="performance" to_port="averagable 1"/>
<portSpacing port="source_model" spacing="0"/>
<portSpacing port="source_test set" spacing="0"/>
<portSpacing port="source_through 1" spacing="0"/>
<portSpacing port="sink_averagable 1" spacing="0"/>
<portSpacing port="sink_averagable 2" spacing="0"/>
</process>
</operator>
<operator activated="true" class="apply_model" compatibility="7.2.000" expanded="true" height="82" name="Apply Model" width="90" x="246" y="34">
<list key="application_parameters"/>
</operator>
<operator activated="true" class="performance_regression" compatibility="7.2.000" expanded="true" height="82" name="Evaluation (2)" width="90" x="380" y="34">
<parameter key="root_mean_squared_error" value="false"/>
<parameter key="absolute_error" value="true"/>
<parameter key="normalized_absolute_error" value="true"/>
<parameter key="squared_error" value="true"/>
</operator>
<operator activated="true" class="log" compatibility="7.2.000" expanded="true" height="82" name="Log" width="90" x="246" y="187">
<list key="log">
<parameter key="C" value="operator.Training.parameter.C"/>
<parameter key="Test Error" value="operator.Validation.value.performance"/>
<parameter key="Training Error" value="operator.Evaluation (2).value.performance"/>
</list>
</operator>
<connect from_port="input 1" to_op="Validation" to_port="training"/>
<connect from_op="Validation" from_port="model" to_op="Apply Model" to_port="model"/>
<connect from_op="Validation" from_port="training" to_op="Apply Model" to_port="unlabelled data"/>
<connect from_op="Validation" from_port="averagable 1" to_op="Log" to_port="through 1"/>
<connect from_op="Apply Model" from_port="labelled data" to_op="Evaluation (2)" to_port="labelled data"/>
<connect from_op="Log" from_port="through 1" to_port="performance"/>
<portSpacing port="source_input 1" spacing="0"/>
<portSpacing port="source_input 2" spacing="0"/>
<portSpacing port="sink_performance" spacing="0"/>
<portSpacing port="sink_result 1" spacing="0"/>
</process>
</operator>
<connect from_op="Generate Data" from_port="output" to_op="Add Noise" to_port="example set input"/>
<connect from_op="Add Noise" from_port="example set output" to_op="ParameterOptimization" to_port="input 1"/>
<connect from_op="ParameterOptimization" from_port="performance" to_port="result 1"/>
<connect from_op="ParameterOptimization" from_port="parameter" to_port="result 2"/>
<portSpacing port="source_input 1" spacing="0"/>
<portSpacing port="sink_result 1" spacing="0"/>
<portSpacing port="sink_result 2" spacing="0"/>
<portSpacing port="sink_result 3" spacing="0"/>
</process>
</operator>
</process>Cheers,
Ingo
2 -
But, hey, you know my opinion of training error by now so don't expect me to be excited about this
What is your opinion Ingo?
0 -
That you should never even think about training error, because it is worse than useless :smileyhappy:
It's not just @IngoRM that thinks that way, but lots of data scientists. With the built-in validation tools that RapidMiner provides to make it easy, you don't ever really care about the training error, just the testing error.
1 -
You bet!
Training error in conjunction with overfitting is still great for selling results to public ???
1 -
:smileylol:
0