Evolutionary Parameter Optimization, bagging and W-RepTree
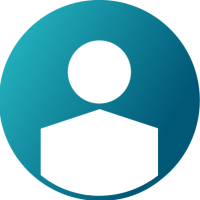
s-a-s-h
New Altair Community Member
Hallo together,
I´ve tried to optimize of a bagging scheme combined with W-RepTree by a parameter optimization. Optimization regarding the Bagging (iterations, sample_ratio) works fine. Optimization of the W-RepTree unfortunately works not with the code below.
Does anybody have advice ?
Thank you,
Sascha
I´ve tried to optimize of a bagging scheme combined with W-RepTree by a parameter optimization. Optimization regarding the Bagging (iterations, sample_ratio) works fine. Optimization of the W-RepTree unfortunately works not with the code below.
Does anybody have advice ?
Thank you,
Sascha
<operator name="Root" class="Process" expanded="yes">
<operator name="ExcelExampleSource" class="ExcelExampleSource">
<parameter key="create_label" value="true"/>
<parameter key="excel_file" value="D:\Dissdaten\Literatur\Papers\ab08-08\DataMiningSoftware\RapidminerYALE\Trainingsdaten5.xls"/>
<parameter key="first_row_as_names" value="true"/>
</operator>
<operator name="EvolutionaryParameterOptimization" class="EvolutionaryParameterOptimization" expanded="yes">
<list key="parameters">
<parameter key="Bagging2.iterations" value="[5.0;100.0]"/>
<parameter key="Bagging2.sample_ratio" value="[0.0;1.0]"/>
<parameter key="Training.M" value="[-1.0;5.0]"/>
</list>
<operator name="Validation" class="XValidation" expanded="yes">
<parameter key="sampling_type" value="shuffled sampling"/>
<operator name="Bagging2" class="Bagging" expanded="yes">
<parameter key="iterations" value="64"/>
<parameter key="sample_ratio" value="0.8633563476940593"/>
<operator name="Training" class="W-REPTree">
<parameter key="L" value="-10.0"/>
<parameter key="M" value="2.387723832548856"/>
<parameter key="N" value="5.0"/>
<parameter key="P" value="true"/>
<parameter key="S" value="56.0"/>
</operator>
</operator>
<operator name="ApplierChain" class="OperatorChain" expanded="yes">
<operator name="Test" class="ModelApplier">
<list key="application_parameters">
</list>
</operator>
<operator name="Evaluation" class="RegressionPerformance">
<parameter key="absolute_error" value="true"/>
<parameter key="main_criterion" value="absolute_error"/>
<parameter key="normalized_absolute_error" value="true"/>
<parameter key="root_mean_squared_error" value="true"/>
<parameter key="squared_error" value="true"/>
</operator>
</operator>
</operator>
<operator name="Log (2)" class="ProcessLog">
<parameter key="filename" value="paraopt.log"/>
<list key="log">
<parameter key="sample_ratio" value="operator.Bagging2.parameter.keep_example_set"/>
<parameter key="M" value="operator.Training.parameter.M"/>
<parameter key="V" value="operator.Training.parameter.V"/>
<parameter key="N" value="operator.Training.parameter.N"/>
<parameter key="S" value="operator.Training.parameter.S"/>
<parameter key="P" value="operator.Training.parameter.P"/>
<parameter key="L" value="operator.Training.parameter.L"/>
<parameter key="absolute" value="operator.Evaluation.value.absolute_error"/>
<parameter key="iterations" value="operator.Bagging2.parameter.iterations"/>
</list>
</operator>
</operator>
Tagged:
0
Answers
-
an extension: the same problems appear with W-FT of the WEKA package...0
-
Hi Sascha,
the problem is within the weka learner W-REPTree. It defines the parameter M as real, but needs an integer. Unfortunatly we have no influence on the weka parameters.
An solution would be to remove the M value from evoultionary parameter optimization and sourround the evolutionary optimization with a gridParameterOptimization. Then you could only insert integer values.
Greetings,
Sebastian0