"Creating SVM learning sets"
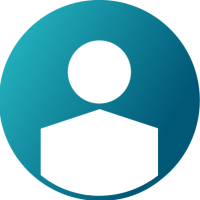
Legacy User
New Altair Community Member
I think I initially put this message in the wrong category, so here it is again:
Hi,
I've been trying to apply SVM on a batch of textual documents in order to evaluate the performance of a model I developed as part of my thesis. First I used the 01_TextClassificationXVal.xml example found in the text plugin documentation. The XML of this example is brought here (I deleted some of the text processing operators - which are irrelevent to my question - in order to make it smaller):
<operator name="Root" class="Process" expanded="yes">
<description text="#ylt#h3#ygt#Optimizing vector creation for text classification#ylt#/h3#ygt##ylt#p#ygt#This experiments shows how to apply a cross validation to a classifier that learns to separate two sets of texts.#ylt#/p#ygt#"/>
<operator name="TextInput" class="TextInput" expanded="yes">
<parameter key="create_text_visualizer" value="true"/>
<list key="namespaces">
</list>
<parameter key="prune_below" value="3"/>
<list key="texts">
<parameter key="graphics" value="../data/newsgroup/graphics"/>
<parameter key="hardware" value="../data/newsgroup/hardware"/>
</list>
</operator>
<operator name="XValidation" class="XValidation" expanded="yes">
<parameter key="leave_one_out" value="true"/>
<operator name="LibSVMLearner" class="LibSVMLearner">
<list key="class_weights">
</list>
<parameter key="kernel_type" value="linear"/>
<parameter key="shrinking" value="false"/>
</operator>
<operator name="OperatorChain" class="OperatorChain" expanded="yes">
<operator name="ModelApplier" class="ModelApplier">
<list key="application_parameters">
</list>
</operator>
<operator name="BinominalClassificationPerformance" class="BinominalClassificationPerformance">
<parameter key="AUC" value="true"/>
<parameter key="f_measure" value="true"/>
</operator>
</operator>
</operator>
</operator>
The problem I have with his example is that the smallest learning set I can use is half of the entire dataset (if I set the value of the cross validation to 2). I would like to use a tenth of the dataset for this purpose, as it is quite large. Is there an operator that can do that for me?
Thanks in advance,
Gil
Hi,
I've been trying to apply SVM on a batch of textual documents in order to evaluate the performance of a model I developed as part of my thesis. First I used the 01_TextClassificationXVal.xml example found in the text plugin documentation. The XML of this example is brought here (I deleted some of the text processing operators - which are irrelevent to my question - in order to make it smaller):
<operator name="Root" class="Process" expanded="yes">
<description text="#ylt#h3#ygt#Optimizing vector creation for text classification#ylt#/h3#ygt##ylt#p#ygt#This experiments shows how to apply a cross validation to a classifier that learns to separate two sets of texts.#ylt#/p#ygt#"/>
<operator name="TextInput" class="TextInput" expanded="yes">
<parameter key="create_text_visualizer" value="true"/>
<list key="namespaces">
</list>
<parameter key="prune_below" value="3"/>
<list key="texts">
<parameter key="graphics" value="../data/newsgroup/graphics"/>
<parameter key="hardware" value="../data/newsgroup/hardware"/>
</list>
</operator>
<operator name="XValidation" class="XValidation" expanded="yes">
<parameter key="leave_one_out" value="true"/>
<operator name="LibSVMLearner" class="LibSVMLearner">
<list key="class_weights">
</list>
<parameter key="kernel_type" value="linear"/>
<parameter key="shrinking" value="false"/>
</operator>
<operator name="OperatorChain" class="OperatorChain" expanded="yes">
<operator name="ModelApplier" class="ModelApplier">
<list key="application_parameters">
</list>
</operator>
<operator name="BinominalClassificationPerformance" class="BinominalClassificationPerformance">
<parameter key="AUC" value="true"/>
<parameter key="f_measure" value="true"/>
</operator>
</operator>
</operator>
</operator>
The problem I have with his example is that the smallest learning set I can use is half of the entire dataset (if I set the value of the cross validation to 2). I would like to use a tenth of the dataset for this purpose, as it is quite large. Is there an operator that can do that for me?
Thanks in advance,
Gil
0
Answers
-
Hi Gil,
whats about sample your data? If I got you right, you don't want to use all your examples for learning. Perhabs you could a sampling algorithm for discarding that portion of data?
Greetings,
Sebastian0 -
Hi Land,
Thanks for answering so quicklly.
You are right - I want to use only a small part of my set for learning, a much smaller part than what is offered by cross-validation. However, I don't know how to apply a sampling algorithm for a TextInput operator. Will it be possible for you (or anyone else, for that matter) to post an example how do do this?
In an attemp to overcome this problem from a different direction, I wrote a java code that can go over all the documents of my dataset and randomly create subsets, which I intended to use as learning sets. I then wrote two simple experiments - one for creating a model based on the subsets I created, and another one that loads that model and applies it one the entore dataset.
In order to make sure these two experiments function properly, I used half the dataset as the learning set (I thought this way I could compare my results to those pruduced by a 2-fold cross validation). Sadly, the results I got were much poorer than those produced by the cross-validation experiment - and I can't understand why that is the case. The XML of the two experiments is posted below - if I made a mistake, please help me understand what it is.
If someone could help me solve even one of these two problems, I think it will be all I need.
Thanks in advance,
Gil
The Two experiments:
1) The learning phase - creating the SVM model:
<?xml version="1.0" encoding="windows-1252"?>
<process version="4.1">
<operator name="Root" class="Process" expanded="yes">
<operator name="TextInput" class="TextInput" expanded="no">
<parameter key="create_text_visualizer" value="true"/>
<list key="namespaces">
</list>
<parameter key="prune_below" value="3"/>
<list key="texts">
<parameter key="type1" value="D:\exp\type1_learnign_set"/>
<parameter key="type2" value="D:\exp\type2_learnign_set"/>
</list>
<operator name="StringTokenizer" class="StringTokenizer">
</operator>
<operator name="EnglishStopwordFilter" class="EnglishStopwordFilter">
</operator>
<operator name="TokenLengthFilter" class="TokenLengthFilter">
<parameter key="min_chars" value="3"/>
</operator>
<operator name="PorterStemmer" class="PorterStemmer">
</operator>
<operator name="TermNGramGenerator" class="TermNGramGenerator">
</operator>
</operator>
<operator name="LibSVMLearner" class="LibSVMLearner">
<list key="class_weights">
</list>
<parameter key="kernel_type" value="linear"/>
</operator>
<operator name="ModelWriter" class="ModelWriter">
<parameter key="model_file" value="C:\Documents and Settings\Admin\Desktop\SVM_Model.mod"/>
</operator>
</operator>
</process>
2) The test phase - applying the model
<?xml version="1.0" encoding="windows-1252"?>
<process version="4.1">
<operator name="Root" class="Process" expanded="yes">
<operator name="TextInput" class="TextInput" expanded="no">
<parameter key="create_text_visualizer" value="true"/>
<list key="namespaces">
</list>
<parameter key="prune_below" value="3"/>
<list key="texts">
<parameter key="type1" value="D:\exp\type1_full_set"/>
<parameter key="type2" value="D:\exp\type2_full_set"/>
</list>
<operator name="StringTokenizer" class="StringTokenizer">
</operator>
<operator name="EnglishStopwordFilter" class="EnglishStopwordFilter">
</operator>
<operator name="TokenLengthFilter" class="TokenLengthFilter">
<parameter key="min_chars" value="3"/>
</operator>
<operator name="PorterStemmer" class="PorterStemmer">
</operator>
<operator name="TermNGramGenerator" class="TermNGramGenerator">
</operator>
</operator>
<operator name="ModelLoader" class="ModelLoader">
<parameter key="model_file" value="C:\Documents and Settings\Admin\Desktop\SVM_Model.mod"/>
</operator>
<operator name="ModelApplier" class="ModelApplier">
<list key="application_parameters">
</list>
</operator>
<operator name="BinominalClassificationPerformance" class="BinominalClassificationPerformance">
<parameter key="AUC" value="true"/>
<parameter key="f_measure" value="true"/>
</operator>
</operator>
</process>
0 -
Hi Gil,
well there is no direct and easy way to execute a cross validation but to use say only 10% of the examples for training and the other 90% for testing purposes. The easy-to-accomplish option you have is to simply use a sampling operator (e.g. [tt]StratifiedSampling[/tt]) before a cross validation. Therewith you may simply discard perhaps about 50% of your data and do a "normal" cross validation on the remaining 50%.
Otherwise you can nearly simulate a kind of multiple validation by the following process:
Note, however, that the examples are not partitioned in the iterations.
<operator name="Root" class="Process" expanded="yes">
<operator name="NominalExampleSetGenerator" class="NominalExampleSetGenerator">
</operator>
<operator name="ParameterIteration" class="ParameterIteration" expanded="yes">
<parameter key="keep_output" value="true"/>
<list key="parameters">
<parameter key="SimpleValidation.local_random_seed" value="1,2,3,4,5,6,7,8,9,10"/>
</list>
<operator name="SimpleValidation" class="SimpleValidation" expanded="yes">
<parameter key="local_random_seed" value="10"/>
<operator name="NaiveBayes" class="NaiveBayes">
</operator>
<operator name="OperatorChain" class="OperatorChain" expanded="yes">
<operator name="ModelApplier" class="ModelApplier">
<list key="application_parameters">
</list>
</operator>
<operator name="Performance" class="Performance">
</operator>
</operator>
</operator>
</operator>
<operator name="AverageBuilder" class="AverageBuilder">
</operator>
</operator>
Regards,
Tobias0