Model Order Reduction of a Multi-scale PBM-DEM Description of a Wet Granulation Process via ANN
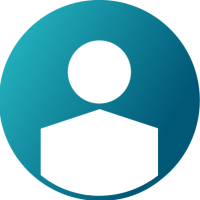
AUTHOR(S)
A. Tamrakar, D. Barrasso, R. Ramachandran
PUBLISHER
Elsevier
SOURCE
Procedia Engineering
YEAR
ABSTRACT
Wet granulation is a particle design process, often used in the pharmaceutical, consumer product, food, and fertilizer industries. A better process understanding is needed to improve process design, control, and optimization. Two modeling frameworks are available to simulate granulation processes: population balance modeling (PBM) and discrete element methods (DEM). PBM simulates changes in the number of particles in each size class due to rate processes such as aggregation, often relying on empirical rate kernels or require additional mechanistic information, such as flux data, collision frequencies, and impact forces. DEM tracks each particle individually, with the abilities to simulate spatial variations and collect mechanistic data. DEM does not inherently simulate particle size changes and is highly computationally expensive. While DEM can determine collision rates between particles of various sizes, PBM can use this data to determine aggregation rates and calculate a net change in the number of particles in each size class. As the size distribution develops, the collision rates change, resulting in a time- and size-dependent aggregation rate kernel. To solve this complex model, reduced order modeling (ROM) is used to replace the computationally expensive DEM step. An artificial neural network (ANN) was trained using DEM results to relate particle size, size distribution, and impeller speed to the collision frequency. Results showed a high correlation between the trained ANN predictions and DEM-generated data. The ANN was coupled with a PBM as a key component of the aggregation rate kernel. The coupled model showed a different development of average particle size and size distribution over time from that of a constant aggregation rate kernel. In addition, the coupled model demonstrated sensitivity to the impeller speed via the ANN rate kernel.
KEYWORDS
Artificial neural network, Discrete element modeling, Population balance modeling, Reduced order modeling, Wet granulation