Fast Design Prediction for Casting Manufacturing Using Physics AI
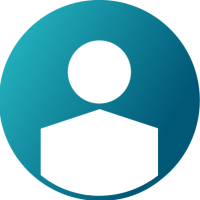

Introduction
One of the most common problems affecting the functionality of the casting component is Porosity. Although it is not possible to achieve zero porosity in the die-casting process, with well-planned mold design and process control you can minimize it. Each process has its method for removing/reducing porosity. The most popular methods for controlling porosity are X-rays, cutting the part, and computerized tomography. Porosity defects occur due to Trapped Gas, Insufficient feeding during solidification, Improper Venting, Gate size, etc.
Problem Statement
Inspire Cast + Physics AI Workflow
Step 1: Gathering historical data of casting simulation performed in the past. Access the h3d files of Filling or Solidification for training purposes. The below image shows the variable used to create various design iterations for the high-pressure die-casting process simulation.
a
Step 2: Create a data set for train and testing inside Physics AI. Train Machine Learning algorithm of Physics AI using historical data from Inspire Cast. Define training variables like depth, width, epochs, and a result type that needs to be trained.
Step 3: Test untrained data against trained data to see the predictive accuracy of the Machine learning algorithm. Validate the ML model against the solver through MAE (Mean absolute error). That explains the predictive accuracy of Physics AI.
Step 4: Import a new design after the satisfactory MAE is achieved. New design import should be similar in terms of mesh and units for accurate predictions. Meshed FEM solver input files can be used to import and predict. Before predicting the new design, make sure to activate the machine learning model that is already trained. Then predict a new design.
Now, all new designs can be predicted faster and without running traditional casting simulations.
Summary: Physics AI is a great tool for fast predictions based on design changes. However, the result's accuracy depends upon the user's trained data set. If the data is not correctly trained, new design predictions won't show promises on result accuracy. So, it is better to understand the type of data being used for training purposes. Below is an example of a new design prediction against actual casting simulation results.