Can I trust the prediction at the new design?
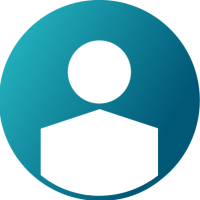

One of the most important questions that needs to be answered with machine learning (ML) models is how confident we are at the prediction for the new design. Hyperstudy uses Quality index to answer this question. The Quality index is provided as a measure to assess both the accuracy and trust in the ML model (Fit) at a specific point in the design space (Fig.1 and 2). Both global and local metrics are combined to create a metric that runs between 0 and an Upper Bound limited by the Fit’s R^2 value. The quality will be highest at points inside the convex hull formed around the Fit’s input points, where the predictive model has been trained to explain variance in the data. The quality metric decreases proportional to the distance outside the convex hull as the predictions at these points becomes less reliable, partially due to the values increasing based on an extrapolation of the data. In simpler terms, the farther your new design is from the training dataset space, the less confident you should be in the prediction (Fig. 3 and 4).
Fig. 1. Quality of the New Design
Fig. 2. Check the Fit Quality to Display the Fit Quality Curve
Fig. 3. Quality of the New Design for a range of Diameter Values
Fig. 4. Lack of Quality on Extrapolated New Designs (Height and Cost Rim Manufacturing variable values are outside the design ranges)