Data Cleansing with Turbo Prep in RapidMiner
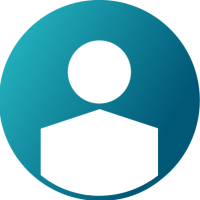
One of the most critical tasks where Data Scientist spends ~40% of their time is Data cleaning. The advantage with Turbo Prep of RapidMiner is that Data Scientist can see how the data looks like after each preparation step. Whether you want to change the name of a column in RapidMiner or delete a column or generate a new column, those tasks could easily be performed by drag and drop using RapidMiner. Also, one could use history to look at what has been done so far and ultimately roll back. In case you want to review, one can go back to the process window in the design panel.
One could easily navigate to the statistics of each column of the dataset. Also, right clicking on the column, would help to go to different options such as Data Transformation, Data Cleansing etc.
Data Cleansing is also one of the key steps in RapidMiner. When we click on a particular column, we find on the left panel different options such as replacing the missing values, dummy encoding or removing the duplicates are activated. Dummy encoding supports conversion of categorical column into numerical column.
Remove Correlated is another important feature, as it helps to reduce overfitting in the data. Once the correlation threshold is set, any columns with higher correlation than the threshold would be removed.
Auto cleansing is another important feature of RapidMiner. The target variable could be selected, which we do not want to be included in Data cleansing. We would also get a preview of the columns from which columns would be excluded.
RapidMiner also offers options in terms of performing PCA or performing Normalization to the end users. The options of manually cleansing the data as well as Auto Cleaning makes RapidMiner a very powerful tool for Data Scientist. Feel free to explore RapidMiner tool, as this tool is free to download for students.