Optimizing electronics manufacturing processes using Altair EDEM simulations
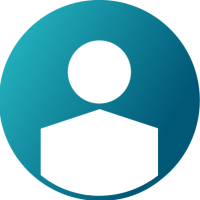

Altair EDEM can simulate the behavior of functional metal powders as well as particle based electromagnetic and conductive coatings, slurries, pastes, and fillers in electronics manufacturing operations. EDEM simulates particulate materials at the particle scale, providing unparalleled insight into process mechanics and enabling the rapid optimization of feedstock properties and process parameters. Leveraging EDEM for virtual prototyping and optimization can reduce reliance on physical testing, shorten development timelines, and result in cost savings.
This article discusses the use of EDEM simulation for electronic component manufacturing through examples for the ceramic capacitor manufacturing process chain and the optimization of slurry rheology.
Simulating ceramic capacitor manufacturing with EDEM
EDEM can be used to simulate key steps in the Multilayer Ceramic Capacitor (MLCC) manufacturing process summarized in Figure 1, including ball milling, mixing, tape-casting, cutting, screen printing, lamination and thermal processing.
|
Figure 1: Schematic diagram of MLCCs and their fabrication process [1]. Reproduced with permission, © The Royal Society of Chemistry
1. Ball milling
Ball milling has been extensively simulated with EDEM to predict and optimize milling efficiency, reduce liner wear, and inform operation and scale-up [2–4]. An example is show below. The particle-particle and particle-liner collisional energy distributions can be analyzed to asses milling performance and liner wear. Process parameters such as rotational velocity, level of fill and ball volume fraction can be virtually optimized to improve milling efficiency and extend liner operational life.
For further details please see:
Breakage and fracture modelling with EDEM
2. Mixing
EDEM simulation can provide unparalleled insight into the mechanics of particulate solid and slurry mixing systems including the internal flow pattern and the spatial-temporal evolution of mixture uniformity. The motion and design of agitators and vessels, as well the method and level of fill, can be virtually optimized to maximize convection in the system, eliminate dead zones and improve efficiency. Virtual trials at varying scales can be performed to inform scale-up, accelerate process design and reduce risks of process failure.
For further details please see:
Analysis and optimisation of bulk solids mixing systems with EDEM
Optimizing a Continuous Mixing Process with Discrete Element Modeling and Machine Learning
3. Tape-casting
Powder and slurry deposition processes such as tape-casting can be simulated in EDEM to predict final layer thickness, surface uniformity, porosity distribution, and particle scale forces. An example is shown below.
4. Screen-printing
EDEM can simulate the flow of particulate solids, pastes and slurries in spreading processes such as screen-printing in order to predict the final porosity distribution and resulting electro-thermal properties of the printed electrode. This enables the virtual optimization of process parameters and feedstock rheology for improved manufacturing accuracy and reliability.
For further details please see:
EDEM Simulation of Powder Spreading in Additive Manufacturing
5. Lamination
High pressure compaction processes such as the lamination process can be simulated in EDEM to understand the compaction mechanics, identify under-consolidated regions and predict the final porosity distribution. The stress on embedded components and their displacement can also be analyzed to assess the likelihood of structural failure or loss of function of the compacted part.
6. Dicing/Cutting
EDEM can simulate the interaction between a rigid blade and a particulate solid, slurry or paste in processes like sheet cutting. The blade velocity and blade geometry can be virtually optimized to minimize local sheet deformation as well as fouling due to material adhesion and dispersion. This can result in improved product quality and reduced process downtime.
7. Thermal processing
EDEM can simulate the conductive, radiative and convective heat flux through a particle assembly arising from a variable temperature heat source. The resulting spatial and temporal evolution of particle temperature, thermo-mechanical and thermo-electric properties can be predicted. It is also possible to capture the shrinkage or expansion of particles during drying and sintering processes to predict the final shape and volume of a particle assembly as well as any residual stresses and fatigue accumulation due to thermal cycling. The simple examples below demonstrate some of the available physics.
Optimizing slurry and paste viscosity with EDEM
Formulating dense suspensions such and slurries and pastes for optimal viscosity is key to meeting product quality requirements in batteries and electronics manufacturing where these materials are a common feedstock or intermediate product (e.g. electrolyte and ceramic slurries, filler pastes etc). However, the traditional physical trial-and-error formulation approach is time consuming and expensive. Formulating virtually using particle scale simulation in Altair EDEM and machine learning in Altair HyperStudy can lead to significant time and costs savings.
Relating the particle properties to the macro-scale properties of powders, slurries and pastes is possible using EDEM simulations of a small volume of material, subjected to an idealized stress or deformation tensor as illustrated in Figure 2.
Figure 2: Relating particle scale to macro-scale properties using EDEM.
Dense particle suspensions can be modeled in EDEM by reducing the particle-fluid interactions to short-range hydro-dynamic forces and fluid drag forces. Using an EDEM model alone also requires the assumption of a constant fluid velocity field and laminar flow conditions throughout the system. This methodology is valid for dense suspensions with a solid fraction higher than 0.45 undergoing steady state deformations in the quasi-static flow regime [5]. The discrete pair-wise particle interactions are described by a viscous hydro-dynamic force model at small separation distances and by an elastic-frictional model during surface-to-surface contact.
The dynamic viscosity of the suspension can be computed by imposing Couette flow in a small periodic cell and integrating the hydro-dynamic, fluid drag, and surface-to-surface contact stresses. An example periodic cell simulation and the corresponding dynamic viscosity results are shown in Figure 3.
Figure 3: EDEM periodic cell simulation for dynamic viscosity determination.
The particle size distribution, shape, volume fraction and contact mechanics can be parameterized in Altair HyperStudy using the EDEM-HyperStudy connector. An example parameter space is shown in Figure 4.
Figure 4: Example parameterization of EDEM particle properties via HyperStudy
The optimal parameter values for a target rheology can then be identified by automatically generating and running EDEM simulations in a Design of Experiments (DoE) configuration, training a Reduced Order Model (ROM) on the data using machine learning, and employing a multi-objective genetic algorithm to rapidly estimate the globally optimal parameter set from the ROM. The optimization methodology is summarized in Figure 5. Leveraging machine learning and optimization in HyperStudy results in several orders of magnitude reduction in computational expense relative to a purely EDEM simulation-based approach, making the virtual optimization of dense suspension rheology from particle scale properties rapid and efficient.
Figure 5: Overview of particle properties optimization with EDEM and HyperStudy
An example outcome of the optimization is the Pareto front of optima shown in Figure 6, where each point corresponds to a unique parameter set that produces the minimum viscosity for a given solid fraction.
Figure 6: Example result from a multi-objective optimization with two competing objectives
Contacts
We are happy to discuss your questions or needs!
Please get in touch at: customer-support@altair.com
References
[1] Hong K, Lee TH, Suh JM, et al. Perspectives and challenges in multilayer ceramic capacitors for next generation electronics. J Mater Chem C 2019, 7: 9782–9802.
[2] M. Mhadhbi, Effect of Milling Parameters on DEM Modeling of a Planetary Ball Mill, Adv. Mater. Phys. Chem. 13 (2023) 49–58. https://doi.org/10.4236/ampc.2023.134004.
[3] K.C. Kim, T. Jiang, N. Il Kim, C. Kwon, Effects of ball-to-powder diameter ratio and powder particle shape on EDEM simulation in a planetary ball mill, J. Indian Chem. Soc. 99 (2022) 100300. https://doi.org/10.1016/j.jics.2021.100300.
[4] S.S. S Paramanantham, B. Brigljević, N. Aleksey, V.M. Nagulapati, G.F. Han, J.B. Baek, H. Mikulčić, H. Lim, Numerical simulation of ball milling reactor for novel ammonia synthesis under ambient conditions, Energy 263 (2023). https://doi.org/10.1016/j.energy.2022.125754.
[5] Cheal, O. and Ness, C. (2018) ‘Rheology of dense granular suspensions under extensional flow’, Journal of Rheology, 62(2), pp. 501–512. doi: 10.1122/1.500400.