Combining EDEM with romAI-An efficient approach to process, product, and workflow optimization
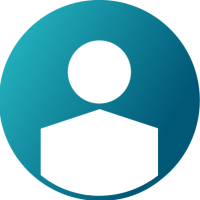

1.0 Introduction
Discrete Element Method (DEM) simulations offer excellent insights into bulk material behavior under various operating conditions and find application in many industries like pharmaceuticals, agriculture, construction and mining, etc.
Explore EDEM applications through webinars
However, such high-fidelity simulations are accompanied by significant computation expenses. romAI offers a robust way to address this challenge through concepts from Reduced Order Modeling (ROM) and Artificial Intelligence (AI). A generic workflow for EDEM-romAI is highlighted in Figure 1. The central idea is to use a physics-based solver to generate training data for a physics-informed neural network.
Figure 1: Generic workflow of combining EDEM and romAI for optimization
2.0 Process Optimization
Process optimization aims to evaluate the correct set of parameter combinations that either minimizes, maximizes, or establishes a reasonable trade-off between various KPIs. The use of EDEM to carry out these iterations can be computationally expensive. Therefore, the approach here is to use a limited number of EDEM runs to create training data for romAI. Once the romAI model is trained and tested, it can be leveraged to sweep across the parameter space to arrive intelligently at an optimal.
This parameter sweep contains far more parameter combinations than the original training data, and thanks to the romAI data driven approach, predicting the response is significantly faster than a physics-based counterpart.
The following are a few examples highlighting such a workflow.
2.1 Design of wheel loader components
A wheel loader is an earth-mover equipment that interacts with bulk materials through a front-mounted bucket. This bucket is maneuvered through hydraulic actuators, allowing for the transport of bulk materials. During service, several components of the wheel loader are subjected to high stresses. This study demonstrates that with a limited number of training runs (generated through coupled EDEM-MotionSolve simulation), a romAI model could be trained with sufficiently high accuracy, which could predict the stress values in the critical components under various sets of handling maneuvers of the front bucket.
Further Details
(1) Using AI and EDEM in Machinery Design: Webinar
(2) How to speed-up DEM simulations leveraging Artificial Intelligence
2.2 Blending of Pharmaceutical Powders
Blending is a key unit operation in the pharmaceutical industry. Ingredients such as excipients and Active Pharmaceutical Ingredients (APIs) are introduced into a bin blender at specified mass fractions and mixed through vessel rotation. This study considers the blender's fill occupancy, axis of rotation, and rotational speed as inputs and monitors the time evolution of the Segregation Index as output.
Using nine EDEM runs as training data, the romAI model was created to sweep across the parameter space to search for an optimal. While the training runs from EDEM take 'days' to complete, romAI predicts the results in a matter of 'seconds.' This allows for the conceptualization of digital twins for real-time prediction of KPIs.
Further Details
1. Combining AI and Simulation for efficient bulk solids handling optimization
2. Digital Twins of Oral Solid Dose (OSD) Manufacturing Process Development and Deployment
3.0 Efficient Material Model Calibration in DEM
Material model calibration involves a calibration test (experiment), followed by recreating the test results in a similar (or equivalent) DEM simulation. This recreation of results is achieved through iterating material interaction parameters. The objective is to evaluate these parameters that best match the simulation response to the experimental results.
A romAI model can be implemented here to sweep across several combinations of material interaction parameters, suggesting the ones that best match the simulation response to the experiment. The following article considers an FT4 Rheometer test as an example to highlight the workflow. The response of the test is the temporal evolution of force and torque curves, as experienced by the Rheometer blade, at different combinations of friction coefficients.
1. More on material model calibration
2. Efficient Material Model Calibration in EDEM – Methods and Example
3. Efficient Material Model Calibration using EDEM, romAI and HyperStudy
4.0 Learning EDEM and romAI
Learning EDEM and romAI can be approached through multiple resources. These resources include eLearning courses, how-to videos, online tutorials, and community articles. Explore the following links for more details
EDEM Material Modelling: 4 Steps to Accelerate Your Learning
5.0 Conclusion
EDEM and romAI are two complementing technologies where one offers high-fidelity physics-based simulation for the exploration of granular behavior, and the other offers high computing speeds for faster iteration for process optimization. Their synergist integration can provide the best of both to the users, offering unparalleled process insights and shorter lead times.