Harnessing Test Data in Model-Based Development through AI: Discovering the Path to Efficiency and Accuracy
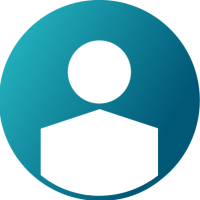

In the rapidly evolving landscape of artificial intelligence (AI), companies are witnessing a shift away from costly, time-consuming activities like prototyping and testing. Instead, they are turning to simulation to optimize processes and meet requirements through the adoption of virtual models. The aim is to design and optimize products quickly and efficiently. However, the key to informed decision-making lies in the deployment of accurate models that can substitute real systems or physical phenomena.
Once virtual models are generated, a crucial step in the process is validating these models through specified proof tests before relying on their outputs. As products become more complex, the needed system-of-systems modeling brings with it numerous nonlinear phenomena and component interactions to consider, which intensifies the challenge of creating accurate virtual prototypes.
Sometimes, traditional equation-based approaches can’t handle precision modeling, particularly when describing nonlinearities in specific phenomena is required, such as damping behavior in mechanical systems or the evaporation temperature in a refrigeration cycle.
The advent of AI presents a groundbreaking solution, facilitating the direct generation of models from real-world data. In this context, test data not only serves for validation but also assumes a pivotal role as the input for the direct creation of models. This approach offers a dual advantage: the rapid, automatic modeling by machine learning algorithms, and the generation of more accurate models, which boast an average accuracy of 98% compared to the 95% accuracy equation-based lumped parameter models typically achieve.
While the use of AI-driven approaches might suggest an increased need for testing that potentially elevates time and cost, this is a false assumption. Modern machine learning algorithms, such as those developed for Altair® romAI™, intelligently utilize data without requiring an excessive number of records. romAI stands out for its ability to generate accurate and efficient static and dynamic models by leveraging existing simulation or test data.
The innovative algorithm it employs aims not only to approximate datasets but to estimate, through data, the system’s underlying mathematics. The result is a model with superior accuracy and generalization capabilities that requires less data compared to other approaches.
Another of romAI’s distinct advantages is its accessibility; its user-friendly interface democratizes access to AI-powered technology to designers, engineers, and technical experts alike. “You don’t need to be a data scientist or programmer to use it,” said Martin Ortega, a design manager at Mabe. “romAI helped us leverage the value of our historical data. Now we are more enabled than ever to continue optimizing our sustainable products and features.” Throughout a recent presentation, Ortega highlighted how romAI enables engineering teams to leverage AI for their core tasks without acquiring coding skills or debugging scripts. Ortega further endorsed romAI for its innovative capability to extract value from historical data, typically siloed and underused within structured organizations, and transform it into a cost-free digital asset.
Want to learn more about romAI and what it can do? Learn more by visiting https://altair.com/romai-applications.