Sobol Sequence: not just another sampling method
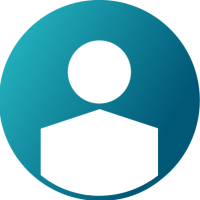

Designs of Experiments (DOE) is a branch of statistics that creates systematic sampling patterns that are used to determine cause and effect relationships between inputs and outputs. DOE is a powerful data collection and analysis tool that can be used in a variety of experimental situations.
Design of Experiments is divided into two main schemes: Factorial and Space-filling designs. Factorial DOEs (Full-Factorial, Fractional Factorial, Taguchi, etc.) provide robust information, but they often require more samples than necessary for exploring cause and effect relationships in highly non-linear systems. Modern space-filling designs are often the most efficient DOEs for simulations and complex physical experiments with highly non-linear responses. And Sobol Sequence is one of those modern methods which is available in HyperStudy as of 2022.3 release and is the theme of this article.
Sobol Sequence belongs to low-discrepancy quasi-random sampling family and provides excellent correlation and uniform distribution performance. The sequence was first proposed by the Russian mathematician Ilya M. Sobol’ in 1967. Each sampling scheme has its own advantages and disadvantages and when it comes to picking the best method, it depends on what capabilities are being looked for. Although, correlation and uniformity performance are typically the decisive aspects, other capabilities may be needed depending on the complexity of the study. For example, for a fixed size of samples, Hammersley and Latin Hypercube can outperform both Modified Extensible Lattice Sequence (MELS) and Sobol based on pure correlation results, as shown in Table 1. However, correlation performance is not the only key factor if extensibility or design variable support or both are sought after.
If sampling is needed for the purpose of building a high-fidelity predictive model, the extensibility of the method can be extremely important as the existing data set may not be sufficient, and more data needs to be added. This is particularly a case in Sampling Fit which relies on extensible sampling strategy. Therefore, the methods available in Sampling Fit are limited to MELS and Sobol. Even though they are both extensible and support design variable constraints, Sobol, depending on the number of dimensions and sample size, may show better correlation performance (see Table 1) and more uniform use of design space as shown in Figure 1.
Data set with uniform distribution plays an important role in calculation of cross-validation R-squared value which is the stopping accuracy metric in Sampling Fit. Sequence in any method defines the pattern of sampled points and the pattern influences overall reliability of R-squared value. In MELS, for example, due to the nature of lattice sequence, points are placed close to each other, and this may lead to biased calculations as validation points are in the proximity of training points. Figure 2 depicts an example of Sampling Fit-based trade-off study with seven design variables and one response targeting 0.95 cross-validation R-squared value. Sampling Fit with MELS takes nine evaluations and with Sobol, it takes thirty-seven runs to reach the target. At first, it seems that using MELS is more efficient since it took only nine runs but as mentioned above, sampling terminated prematurely due to biased calculation of cross-validation R-squared. For the given set of design variable values, predictive model of Sobol Sequence provides more reliable output judging by its higher quality value (less amount of extrapolation). These inferences are drawn based upon a single combination of design variables. For any other given combination, MELS and Sobol may show similar performance.
Sobol has been added to compliment our existing methods and it is an excellent extensible space-filling DOE with great correlation performance.
Two separate study archives pertaining to Figures 1 and 2 are included if you are interested in having a look at them. Should you have any questions and comments, please feel free to leave them below.