Optimizing Parameters of a Structural Analysis Using Artificial Intelligence (AI)
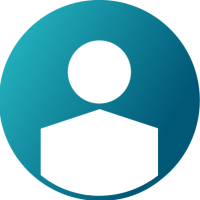

Abstract
In this article we will use a novel AI-based application, romAITM, to generate a Reduced-Order-Model (ROM) from a large database of static simulations and optimize their parameters. We will demonstrate how this innovative approach can support the design of an Aircraft Wing.
The romAI model will be used to estimate the stress and displacement of a critical region varying the thicknesses of the model, obtaining a simulation runtime around 98% faster.
Introduction
On this application we discuss the analysis of an aircraft wing with static loads. This system can be simulated with the aid of Altair’s software. A complex finite element (FE) model can be modeled on HyperWorks®, using different components, materials, contacts, loads and solver it on OptiStruct®.
As expected, different thicknesses in the model vary the stress and displacement. In this case there is a critical region for each of these results that will be monitored to optimize the thicknesses.
The Challenge
Traditionally for each different thickness, we would have to run a simulation on OptiStruct. It generates a large database with several simulation data that could be hard to interpret and get insights in product development.
Besides that, it is worth mentioning that even static simulations using FE can be time costly, particularly on large and complex models.
Therefore, the main objective of this analysis is optimizing the thickness of two sheet metal aiming a desired stress of a critical region using a ROM to get faster results.
Workflow
We’ve followed a 2-step workflow:
- ROM generation & Validation using simulation data
- ROM optimization deployment & results evaluation
(1) The database has more than 300 simulation results to be used in the romAI training.
The csv file with the output data was used in romAI GUI for the generation of the dynamic reduced-order model.
The inputs to the system are the already mentioned (thickness of two different sheet metal) while the outputs are stress and displacement of two different (both critical) regions.
(2) Next, we validate the romAI model by comparing its outputs with the OS ones for the same inputs.
Once we have validated the generated ROM, we create an optimization model that will search for the best thicknesses for a desired stress.
Finally, to have a reference for the comparison, we ran the OptiStruct model with the optimized thicknesses and compare the results.
It is remarkable that the difference on the von Misses stress on the critical regions between the FE simulation and the romAI is under 3%!
Conclusion
A proper reduced-order model was generated starting from data obtained by OptiStruct simulations, covering an adequate range of thicknesses and their results.
The high complexity of the FE model with a non-intuitive load, several contacts and loads model makes this approach even more challenging. Nevertheless, romAI allowed to predict the stress and displacement on critical regions with a small deviation.
- The romAI can estimate the stress in the critical region with high accuracy (difference less than 3%), obtaining a simulation runtime around 98% faster.
Do not hesitate to contact Altair for additional information on System Simulation combined with AI!
Comments
-
Hi João Marabisa,
It is a really interesting test case and the obtained results are promising, is it possible to share the DOE setup for FEA and ROM along with the results?
I'm eager to replicate the entire process, from the CAE setup to the romAI process myself.
Many thanks.
Best
Abhijith
0