Designing Better Products with Machine Learning in HyperWorks
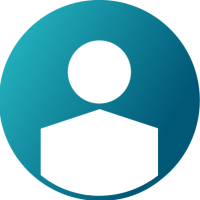

Machine learning and artificial intelligence are increasingly referenced in our everyday lives. The terms have become ubiquitous and as an engineer in the world of simulation, it’s natural to wonder how these technologies will assimilate into our daily work. Low code or no code platforms such as Altair Knowledge Works make the tools of data science accessible to an increasingly wider audience by lowering the barrier of entry. These solutions further the goal of democratizing data science, but their nature still requires familiarity with a collection of abstract plots, charts, and metrics. The Design Explorer workflows in Altair HyperWorks 2021 bring engineers AI insights from the simulated physics directly into their daily working environment. The seamless integrations bring intuitive analytics with only a few clicks.
The full potential of CAE has always been built on the axiom that crashing virtual cars or bending virtual wings is cheaper than the corresponding physical tests. Final verification via physical tests is still required, of course, but only to confirm the predicted outcome of the virtual design process. Through much of its history, CAE had been used primarily as a trial and error process of design improvement. Scalable computing clusters, including most recently cloud environments, allow for simulation on a scale that was previously unimaginable. Machine learning techniques are ideal tools to sift through the mountains of data. An easy to use solution must deliver a user-friendly solution to 3 critical steps of the process.
1 – Define the scope of the problem. The inputs and outputs can be manually defined or automatically collected. Examples include features such as material, geometry, or loading or Key Performance Indices like stress or mass.
2- Collect virtual samples. Keeping track of all the data requires a management system and when data doesn’t yet exist, an integrated simulation and data management system is required to synthesize the data on demand.
3- Provide visual explanations. This piece is key to a natural user experience. I feel the ultimate goal here is most appropriately expressed by Edward Tufte, a leading expert in the field of information design : “Don’t think. Look”.
The Design Exploration workflows satisfy each of the 3 requirements. A dedicated ribbon in the user interface let’s user setup their problem intuitively by clicking directly on the 3d model.
Once the problem scope is well posed, the next step is to manage the simulation and data collection. Creating a comprehensive sampling strategy, executing the series of simulations, and presenting status of the ongoing process is handled within the user interface.
Finally, the user is presented with easily interpretable analytics that let the user focus on the engineering. The influence of an input on the design performance are shown directly on the model, using color and saturation to aid visualization.
New in 2021 is the introduction of full simulation predictions to visualize the impact of design changes in real time. This cutting edge AI learns from the virtual data to give instant insight with no coding or parameter tuning. It is truly automatic machine learning within the reach of any user.
These examples are just the beginning of what we at Altair have called engineering data science. We have many more ideas for the future. #onlyforward
What are your thoughts on how engineering and data science will continue to evolve? Let us know in the comments below.
Comments
-
Very Insightful Joe. EDS it is! I learnt this morning, that on average, for a new car platform to see light, they run anywhere close to 5000 crash simulations with each simulation running 20 hours each. At the end of it all, this data is cleaned up every 6 months. Clearly, its a ski down a mountain if data is synthesized for you and all the KPI's are readily captured before data is thrown away. Design Explorer is a clear winner and has lot of potential applications.
I realized we also have to ability to easily create and manage plenty of virtual samples using our very own Free size and Free shape optimization in OptiStruct. Do you think there is merit in ingesting these shapes and sizes to further field predictions?
2 -
I think the third point that you made is probably the most revolutionary part of the workflow above... Methods and tools to preform Design of Experiments have been around for a while now, but have been relegated to a certain level of expertise that only the engineering gods can bestow upon you when you are deemed worthy. Interpreting results from all of this data took a certain knowledge about math and methods... But now, being able to plot directly on the model, and SEE the effects carries so much more weight and an instant understanding.
I would really be interested in seeing what AI can do with previous designs/analysis. If AI is able to take bits and pieces of certain designs that worked well, and avoid features that caused problems in legacy versions, and combine them together to make the ultimate part without the need for much engineering. I suppose this is some sort of Smart Generative Design, that takes into account all previous designs to only build better and better products.
Both excited and scared to see what AI has in store for the engineering community...
2 -
Rejeesh Rajan_22118 said:
Very Insightful Joe. EDS it is! I learnt this morning, that on average, for a new car platform to see light, they run anywhere close to 5000 crash simulations with each simulation running 20 hours each. At the end of it all, this data is cleaned up every 6 months. Clearly, its a ski down a mountain if data is synthesized for you and all the KPI's are readily captured before data is thrown away. Design Explorer is a clear winner and has lot of potential applications.
I realized we also have to ability to easily create and manage plenty of virtual samples using our very own Free size and Free shape optimization in OptiStruct. Do you think there is merit in ingesting these shapes and sizes to further field predictions?
That is an interesting idea. Looking at using historical FEA data is one of our goals, but specifically using info from free size and shape is an interesting idea.
0 -
I am very interested to see ML & AI deployed across various industry verticals - heavy machinery, biomedical, consumer goods, O&G, aerospace to name just a few. There has been a lot of buzz around these topics and through Design Explorer it really appears to be accessible to a much broader audience of engineers who are not necessarily ML/AI experts.
DE should prove to be of great value as each industry matures their processes further toward the left of the "design Vee" to simulation driven design. Less track time, less bench tests, less clinical tests on animals and humans; more simulation! And with more samples comes more reliability and confidence.
Where can we find tutorials?
0 -
Michael Johnson_21139 said:
I am very interested to see ML & AI deployed across various industry verticals - heavy machinery, biomedical, consumer goods, O&G, aerospace to name just a few. There has been a lot of buzz around these topics and through Design Explorer it really appears to be accessible to a much broader audience of engineers who are not necessarily ML/AI experts.
DE should prove to be of great value as each industry matures their processes further toward the left of the "design Vee" to simulation driven design. Less track time, less bench tests, less clinical tests on animals and humans; more simulation! And with more samples comes more reliability and confidence.
Where can we find tutorials?
Hi Michael,
You can find the DE documentation here. It has a couple examples (tutorials) to get you started.
Here's the link directly to the tutorials.Finally, I'm not sure if it's accessible to all, but here's a Confluence page, which has links to the documentation and tutorials, but also a bunch of other DE-related links.
Please let me know any time if you have questions!
0 -
Blaise Cole_21594 said:
I think the third point that you made is probably the most revolutionary part of the workflow above... Methods and tools to preform Design of Experiments have been around for a while now, but have been relegated to a certain level of expertise that only the engineering gods can bestow upon you when you are deemed worthy. Interpreting results from all of this data took a certain knowledge about math and methods... But now, being able to plot directly on the model, and SEE the effects carries so much more weight and an instant understanding.
I would really be interested in seeing what AI can do with previous designs/analysis. If AI is able to take bits and pieces of certain designs that worked well, and avoid features that caused problems in legacy versions, and combine them together to make the ultimate part without the need for much engineering. I suppose this is some sort of Smart Generative Design, that takes into account all previous designs to only build better and better products.
Both excited and scared to see what AI has in store for the engineering community...
"Design of Experiments have been around for a while now, but have been relegated to a certain level of expertise that only the engineering gods can bestow upon you when you are deemed worthy"
I would re-phrase this as "those who were not bestowed upon by physics gods worked on DoE".
I think it is more exciting than scary. Who wants to labor over meshing, when you can be reviewing parallel coordinate plots to come up with better, robust designs. This gives me an idea for a future Altair Community article!
0