DIY Machine Learning in Altair Simulation Suite is Shockingly Easy
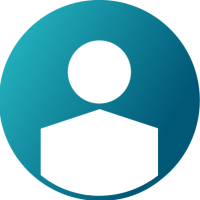

Learning from simulated data has never been easier. Embrace your inner data scientist and include your own favorite algorithm in your next project.
Using math and statistics to draw inferences from experimental data is an old concept. Although he didn’t invent all the math, Ronald Fisher’s early 20th century analysis of agronomy experiments both established and popularized many of the techniques still widely in use today. In the last decades of the 20th century, rising computer power fueled the growth of computer experiments, where complex models and equations are solved to simulate reality. Correspondingly, dedicated software evolved to easily plan, execute, and learn from the synthesized data by simplifying the complexities of process integration, data acquisition, and mathematical analysis into a turnkey solution. Most recently, general interest in machine learning to detect patterns in data is leading to interest beyond the out of the box algorithms prepackaged into software. Modern sophisticated users often look to match their best algorithm to the specifics of their problem.
To be clear, machine learning did not create this sophisticated user, but its popularity has increased their population. Organizations that used to conduct only a few advanced data projects are now looking to repeat their successes broadly. Project customized notebook scripts and one-off user interfaces are inefficient at scale. Plugging custom methods into established user interfaces is an efficient way to develop, test, and deploy machine learning models in your project work.
Altair’s dedicated data analytics software, such as SmartWorks or Knowledge Studio, each feature “bring your own model” functionality. HyperStudy, as an engineering data science software, can integrate user defined supervised learning algorithms to match data science expertise to engineering design and optimization problems.
After a simple registration, your custom methods are available within the HyperStudy interface, right alongside the out of the box algorithm options.
With post-processing visualization already integrated, it is easy to validate your model’s performance via comparisons to out of the box solutions, as shown below in side by side bar charts.
Like a 21st century Ronald Fisher, it is possible to establish and popularize you own methods across your organization. No need to be humble, I’d like to hear about how well your DIY methods perform on your next project.